Master's Thesis : Evaluation and Integration of Deep Learning Architectures for Automatic Defect Recognition
Sprumont, Damien
Promoteur(s) :
Geurts, Pierre
Date de soutenance : 7-sep-2020/9-sep-2020 • URL permanente : http://hdl.handle.net/2268.2/10716
Détails
Titre : | Master's Thesis : Evaluation and Integration of Deep Learning Architectures for Automatic Defect Recognition |
Titre traduit : | [fr] Évalutation et Intégration d'Architectures "Deep Learning" pour la Reconnaissance Automatique de Défauts |
Auteur : | Sprumont, Damien ![]() |
Date de soutenance : | 7-sep-2020/9-sep-2020 |
Promoteur(s) : | Geurts, Pierre ![]() |
Membre(s) du jury : | Marée, Raphaël ![]() Louppe, Gilles ![]() Libertiaux, Vincent |
Langue : | Anglais |
Nombre de pages : | 66 |
Mots-clés : | [en] GDXRay, ADRIC, Deep Learning, Defect detection |
Discipline(s) : | Ingénierie, informatique & technologie > Sciences informatiques |
Public cible : | Chercheurs Professionnels du domaine Etudiants |
Institution(s) : | Université de Liège, Liège, Belgique |
Diplôme : | Master en ingénieur civil en informatique, à finalité spécialisée en "intelligent systems" |
Faculté : | Mémoires de la Faculté des Sciences appliquées |
Résumé
[en] Industrial control has take more and more importance as pieces are often designed to meet
the technical requirements while being the more lighter and cheaper. In the same time, an
operator is responsible for manually inspect and detect defective part from non-defective
ones, which can less and less be achieved in reasonable times for large amount of pieces.
This feeds the demand for innovative and efficient methods to inspect and discriminate
between defective and non-defective parts. With the impulsion of companies like X-RIS,
Euresys and Optrion, the Walloon Region has launched the ADRIC project. This project
aims at developing numeric solutions to face the aforementioned problem. The goals of
this thesis are to investigate deep learning solutions, to implement these solutions and to
evaluate these solutions over x-ray images of industrial pieces. Two datasets are provided
by the ADRIC team and hosted on Cytomine[19]. The GDXray[21] dataset is also used
in the experiments. One evaluates the Mask R-CNN[9] model within the Detectron2[24]
framework. One also builds our own custom models and evaluates those models, to assess
the influence of several parameters on their accuracy. the best model is then integrate
to the Cytomine Research platform. One follows the integration protocol and release an
inference algorithm based on a pre-trained model, previously selected.
Fichier(s)
Document(s)
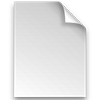

Description: Thesis (core + appendices)
Taille: 3.01 MB
Format: Adobe PDF
Citer ce mémoire
L'Université de Liège ne garantit pas la qualité scientifique de ces travaux d'étudiants ni l'exactitude de l'ensemble des informations qu'ils contiennent.