Master's Thesis : Papyrus matching using machine learning
Nicolas, Pierre-Loup
Promotor(s) :
Geurts, Pierre
;
Marée, Raphaël
Date of defense : 7-Sep-2020/9-Sep-2020 • Permalink : http://hdl.handle.net/2268.2/10719
Details
Title : | Master's Thesis : Papyrus matching using machine learning |
Author : | Nicolas, Pierre-Loup ![]() |
Date of defense : | 7-Sep-2020/9-Sep-2020 |
Advisor(s) : | Geurts, Pierre ![]() Marée, Raphaël ![]() |
Committee's member(s) : | Van Droogenbroeck, Marc ![]() Polis, Stéphane ![]() |
Language : | English |
Discipline(s) : | Engineering, computing & technology > Computer science |
Institution(s) : | Université de Liège, Liège, Belgique |
Degree: | Master en science des données, à finalité spécialisée |
Faculty: | Master thesis of the Faculté des Sciences appliquées |
Abstract
[fr] For papyrologists, the task of reconstructing papyri from scattered fragments is a tedious and time-consuming, yet crucial task to uncover new texts that could provide meaningful information on a variety of subjects.
With the advent of digital humanities, several tools have been developed to make papyrological studies more effective, in particular when it comes to the digitization and manipulation of papyri. But none of these tools can help papyrologists for the decision-making involved in the reconstruction of papyri.
In recent years, it has been shown that deep learning techniques can be successfully applied to a wide variety of problems, sometimes with impressive results. However, the application of such techniques to papyrology is pretty much novel.
In this thesis, deep learning techniques are applied to the task of papyrus fragment matching, to evaluate the possibilities offered by neural networks to tackle this complex task. Using a papyrus fragment dataset built from scratch using raw data from the papyrus collection of the Museo Egizio of Turin, several siamese neural network architectures are evaluated and compared.
The best performing model is also evaluated on more a realistic use case and gives some encouraging results, though it is not yet ready for practical use. Several improvements are proposed to further improve the results.
File(s)
Document(s)
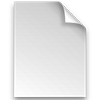

Description:
Size: 23.24 MB
Format: Adobe PDF
Cite this master thesis
APA
Nicolas, P.-L. (2020). Master's Thesis : Papyrus matching using machine learning. (Unpublished master's thesis). Université de Liège, Liège, Belgique. Retrieved from https://matheo.uliege.be/handle/2268.2/10719
Chicago
The University of Liège does not guarantee the scientific quality of these students' works or the accuracy of all the information they contain.