Reconstruction of missing data in HF radar observations using the convolutional autoencoder DINCAE
Houyoux, Aimée
Promoteur(s) :
Barth, Alexander
Date de soutenance : 24-jui-2021/25-jui-2021 • URL permanente : http://hdl.handle.net/2268.2/11517
Détails
Titre : | Reconstruction of missing data in HF radar observations using the convolutional autoencoder DINCAE |
Auteur : | Houyoux, Aimée ![]() |
Date de soutenance : | 24-jui-2021/25-jui-2021 |
Promoteur(s) : | Barth, Alexander ![]() |
Membre(s) du jury : | Beckers, Jean-Marie ![]() Capet, Arthur ![]() Geurts, Pierre ![]() |
Langue : | Anglais |
Mots-clés : | [fr] HF radars, Deep Learning, Autoencoder |
Discipline(s) : | Ingénierie, informatique & technologie > Ingénierie électrique & électronique Physique, chimie, mathématiques & sciences de la terre > Multidisciplinaire, général & autres |
Institution(s) : | Université de Liège, Liège, Belgique |
Diplôme : | Master : ingénieur civil électricien, à finalité spécialisée en "electronic systems and devices" |
Faculté : | Mémoires de la Faculté des Sciences appliquées |
Résumé
[fr] [eng]The goal of this work is to evaluate the possiblility to reconstruct missing surface current datafrom high frequency radars (HFR) with a convolutional neural network. HF radars measure surfacecurrents based on Bragg scattering of electromagnetic waves upon gravity waves on the ocean surface.Perturbation of the process can occur due to interferences or presence of metallic objects in the measuredfield and cause gaps in the collected data.Data-Interpolating Convolutional Autoencoder (DINCAE) is here applied to total currents measure-ments in the Ibiza Channel that are and made freely available by the Balearic Islands Coastal OceanObserving and Forecasting System (SOCIB) since 2012. The structure of the Autoencoder allows thenetwork to be trained on fields with missing data.Both zonal currents (U) and meridional currents (V) are given as inputs to the network are reconstructedalong with the expected standard deviation of the error of reconstruction, following a gaussian curve. Mainpatterns in the data are captured by the encoder part of the network thanks to convolutional filters andpooling and from there, the full fields are reconstructed by the decoder part.During training of the network, a part of the data was set aside to perform cross-validation. Maskswere added to those data to assess the ability of DINCAE to reconstruct the hidden data. Performancesof the reconstruction were computed for different hyperparameter combinations. Presence of drifters inthe area in 2014 further gave the possibility to make a second validation with external data.Since the two validation strategies gave different results from each other, a configuration selected byeach validation was further analysed. It came out that cross-validation selected configuration is presumablytrapped in a local minimum because of a too high lower limit on the expected standard deviation. Infact this is an issue encountered with many tests that were carried out. Drifter selected configuration isthus more reliable and will lead to better generalization, though some time instances are polluted withartefacts, explaining the poor results with cross-validation (performed on a longer time interval).Finally, drifter validation makes comparison with other reconstruction methods possible. DINCAE isfound to make more accurate reconstructions than Open-Boundary Model Analysis (OMA), which is avery commonly used method, but not as good as Data Interpolation Variational Analysis in n-dimensions(DIVAnd).
Fichier(s)
Document(s)
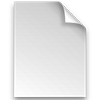

Description: -
Taille: 230.25 kB
Format: Adobe PDF
Annexe(s)
Citer ce mémoire
L'Université de Liège ne garantit pas la qualité scientifique de ces travaux d'étudiants ni l'exactitude de l'ensemble des informations qu'ils contiennent.