Blind Grasping: Object Classification using Robot Joint Information
Schneider, Philippe
Promotor(s) :
Louppe, Gilles
;
Bruls, Olivier
Date of defense : 6-Sep-2021/7-Sep-2021 • Permalink : http://hdl.handle.net/2268.2/13049
Details
Title : | Blind Grasping: Object Classification using Robot Joint Information |
Translated title : | [fr] Grasping aveugle : Classification d'objets à l'aide d'informations sur les articulations du robot |
Author : | Schneider, Philippe ![]() |
Date of defense : | 6-Sep-2021/7-Sep-2021 |
Advisor(s) : | Louppe, Gilles ![]() Bruls, Olivier ![]() |
Committee's member(s) : | Sacré, Pierre ![]() Louveaux, Quentin ![]() |
Language : | English |
Number of pages : | 74 |
Keywords : | [en] Grasping, Deep Learning, Robotics, Machine Learning |
Discipline(s) : | Engineering, computing & technology > Computer science |
Commentary : | promoteur: Prof. Gilles Louppe |
Institution(s) : | Université de Liège, Liège, Belgique |
Degree: | Master en ingénieur civil en informatique, à finalité spécialisée en "intelligent systems" |
Faculty: | Master thesis of the Faculté des Sciences appliquées |
Abstract
[en] Machine learning has proven itself to be a useful tool in many scientific fields. Among the plethora of applications, modern Robotics aims to incorporate artificial intelligence, in order to tackle tasks that were previously unthinkable to be performed by a robot. Grasping is a classic example of such a challenge. Being able to grasp and manipulate a wide range of objects reliably represents a major stepping stone in both productivity and flexibility for modern robots.
The first step of grasping an object is to identify it correctly. To that end, most state of the art approaches to robotic grasping include all kinds of visual input to facilitate the location and identification of the target object. This work aims to investigate a different approach, where object classification is tackled using exclusively the robot's joint information. More specifically this project explores the question: "How useful is robot joint information for the classification of objects?" The main contributions of this work are various benchmark experiments as a proof of concept, additional methods aiming to increase classification performance, as well as the application on a set of real world objects. The results and observations of the different problems settings in this work lead to the conclusion that joint information is indeed useful and exploitable, but also comes with clear limitations and challenges, some of which are easier to circumvent than others.
File(s)
Document(s)
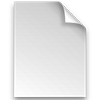

Description: Main report
Size: 20.3 MB
Format: Adobe PDF
Cite this master thesis
APA
Schneider, P. (2021). Blind Grasping: Object Classification using Robot Joint Information. (Unpublished master's thesis). Université de Liège, Liège, Belgique. Retrieved from https://matheo.uliege.be/handle/2268.2/13049
Chicago
The University of Liège does not guarantee the scientific quality of these students' works or the accuracy of all the information they contain.