Master thesis : Depth estimation from sports broadcast videos
Liesse, Valentine
Promotor(s) :
Van Droogenbroeck, Marc
Date of defense : 27-Jun-2022/28-Jun-2022 • Permalink : http://hdl.handle.net/2268.2/14382
Details
Title : | Master thesis : Depth estimation from sports broadcast videos |
Author : | Liesse, Valentine ![]() |
Date of defense : | 27-Jun-2022/28-Jun-2022 |
Advisor(s) : | Van Droogenbroeck, Marc ![]() |
Committee's member(s) : | Barnich, Olivier
Cioppa, Anthony ![]() |
Language : | English |
Number of pages : | 109 |
Keywords : | [en] Deep learning [en] computer vision [en] depth estimation [en] self-supervised [en] monodepth2 [en] stereo vision [en] monocular vision |
Discipline(s) : | Engineering, computing & technology > Computer science |
Institution(s) : | Université de Liège, Liège, Belgique |
Degree: | Master : ingénieur civil électricien, à finalité spécialisée en "signal processing and intelligent robotics" |
Faculty: | Master thesis of the Faculté des Sciences appliquées |
Abstract
[en] Nowadays, broadcasters must continually innovate to create compelling content that evokes emotions in viewers. Photo-realistic depth of field effect, augmented reality, or even 3D reconstruction could be one way to achieve this. Depth estimation is one of the steps to video understanding that allows these applications.
In this master thesis, methods to estimate a depth map from a single image were explored. Especially, deep learning methods were preferred to those based on geometry or the use of sensors. Thus, the solution provided by this work is entirely software-based.
In particular, a custom model has been created for a sports broadcast dataset. However, this dataset does not contain any ground truth depth map. Hence, self-supervised monocular depth estimation methods were investigated in this document. Specifically, trainings were performed with non-rectified and synchronized stereo images captured with uncalibrated cameras. In contrast to trainings based on monocular sequences, the scenes are static and the only movement is that of the camera. This is the ideal configuration for training with self-supervised methods. However, to our knowledge, we are the only ones to have attempted to train self-supervised monocular depth estimation methods using non-rectified stereo images captured with uncalibrated cameras. More precisely, in this work, the intrinsic and extrinsic parameters of the cameras are estimated during training along with the depth map.
During the course of this research, we were able to demonstrate the ability of self-supervised methods to predict coherent depth maps when trained on non-rectified stereo sports broadcast videos captured with uncalibrated cameras. Moreover, we addressed the limitations regarding the generation of depth maps under those conditions.
File(s)
Document(s)
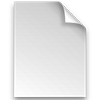

Description:
Size: 172.19 MB
Format: Adobe PDF
Annexe(s)
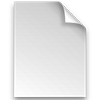

Description:
Size: 1.91 MB
Format: Adobe PDF
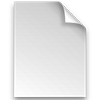

Description:
Size: 174.93 kB
Format: Adobe PDF
Cite this master thesis
The University of Liège does not guarantee the scientific quality of these students' works or the accuracy of all the information they contain.