Master thesis : Super-Resolution Techniques for Broadcast Videos Using Deep Neural Networks
Langer, Guillaume
Promotor(s) :
Louppe, Gilles
Date of defense : 27-Jun-2022/28-Jun-2022 • Permalink : http://hdl.handle.net/2268.2/14399
Details
Title : | Master thesis : Super-Resolution Techniques for Broadcast Videos Using Deep Neural Networks |
Author : | Langer, Guillaume ![]() |
Date of defense : | 27-Jun-2022/28-Jun-2022 |
Advisor(s) : | Louppe, Gilles ![]() |
Committee's member(s) : | Marée, Raphaël ![]() Fontaine, Pascal ![]() Botta, Vincent |
Language : | English |
Discipline(s) : | Engineering, computing & technology > Computer science |
Institution(s) : | Université de Liège, Liège, Belgique |
Degree: | Master : ingénieur civil en science des données, à finalité spécialisée |
Faculty: | Master thesis of the Faculté des Sciences appliquées |
Abstract
[en] With 4K technologies becoming increasingly available, suitable ultra-high definition video content is more and more demanded. Indeed, lower resolution video standards such as Full HD cannot be rendered as is on 4K devices, otherwise visual artifacts appear and decrease the perceptual quality. A possible solution to this problem is to use space-time video super-resolution to convert older Full HD content into new 4K videos for a smoother visual experience.
EVS Broadcast Equipment is a leading company in the broadcast industry. They provide solutions to the most important sports leagues worldwide, and are also active in many other fields. At their request, this work aims to develop a space-time super-resolution method to convert Full HD content to 4K. However, the footage handled at EVS is demanding, with generally fast and large motions and a high level of detail. This aspect is therefore taken into account, as well as the fact that EVS must often work in real time. In addition to have good performance, the developed method is also designed to be as fast as possible.
In this work, an overview of the related literature is first proposed, highlighting the fact that deep neural networks are the state-of-the-art in space-time video super-resolution. However, most methods are developed to produce relatively low resolution content. The current work is based on two of them, which are therefore heavily modified to work on 4K. Furthermore, in addition to building a suitable architecture, a large amount of data is collected to properly train the model. Finally, a highly parallelized inference approach is proposed, and concrete example of 4K videos generated by the developed method are provided.
File(s)
Document(s)
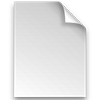

Description: -
Size: 18.9 MB
Format: Adobe PDF
Annexe(s)
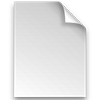

Description: -
Size: 32.12 kB
Format: Adobe PDF
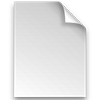

Description: -
Size: 1.14 MB
Format: Adobe PDF
Cite this master thesis
APA
Langer, G. (2022). Master thesis : Super-Resolution Techniques for Broadcast Videos Using Deep Neural Networks. (Unpublished master's thesis). Université de Liège, Liège, Belgique. Retrieved from https://matheo.uliege.be/handle/2268.2/14399
Chicago
The University of Liège does not guarantee the scientific quality of these students' works or the accuracy of all the information they contain.