Master thesis : Large Scale and Real-Time Cattle Behaviour Recognition by Deep Learning on Video Data
Lievens, François
Promotor(s) :
Louppe, Gilles
Date of defense : 27-Jun-2022/28-Jun-2022 • Permalink : http://hdl.handle.net/2268.2/14427
Details
Title : | Master thesis : Large Scale and Real-Time Cattle Behaviour Recognition by Deep Learning on Video Data |
Translated title : | [fr] Reconnaissance à grande échelle et en temps réel des comportements de bovins par l'utilisation de l'apprentissage profond |
Author : | Lievens, François ![]() |
Date of defense : | 27-Jun-2022/28-Jun-2022 |
Advisor(s) : | Louppe, Gilles ![]() |
Committee's member(s) : | Van Droogenbroeck, Marc ![]() Marée, Raphaël ![]() Garnier, Quentin |
Language : | English |
Number of pages : | 96 |
Keywords : | [en] Data Science [en] Deep Learning [en] Livestock [en] Action recognition [en] Computer Vision [en] animal welfare [en] animal health |
Discipline(s) : | Engineering, computing & technology > Computer science |
Commentary : | Travail réalisé en collaboration avec AIHerd |
Funders : | AIHerd |
Target public : | Researchers Professionals of domain Student General public |
Complementary URL : | https://www.aiherd.io/ |
Institution(s) : | Université de Liège, Liège, Belgique |
Degree: | Master en science des données, à finalité spécialisée |
Faculty: | Master thesis of the Faculté des Sciences appliquées |
Abstract
[en] Action recognition on video data is an exploding discipline in the deep learning sector. The consequent increase in available computing power combined with improvements achieved in deep learning methods now allow us to use powerful algorithms on real videos. In the frame of this project, we have worked with AIHerd, a company that designs an intelligent video surveillance tool aiming to improve the management of dairy herds. AIHerd already handle the identification and the tracking of every animals covered by a camera sky. The goal of this project was to add to this architecture a new brick capable of characterizing their behaviours and interactions in real time. We first developed an algorithm for extracting and selecting specific video sequences
of animals performing a potentially interesting behaviour. These data were used to encode short videos hosted on an annotation platform modified to our data. Students were selected and trained to annotate these videos, allowing the creation of an action recognition dataset of behaviours on which our models were trained. A prediction algorithm was implemented in order to manage a large number of possible configurations. These configurations allow us to use strategies from the state of the art in terms of action recognition, but also to evaluate several strategies of our creation to ensure a better trade-off between prediction quality and resource cost. Our training and evaluation algorithms have successfully met the technical challenge of classifying in real time a total of 16 behaviours categories simultaneously on 120 cattle covered by 5 high definition cameras. We also demonstrated that adapting old strategies, combined with various components inspired by the state of the art of deep learning, allows us to obtain an algorithm that is light enough to be integrated into the AIHerd software with a restricted decrease in prediction quality compared to the state of the art methods.
File(s)
Document(s)
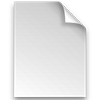

Description: Master Thesis of François Lievens (2022)
Size: 36.29 MB
Format: Adobe PDF
Annexe(s)
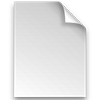

Description: AIHerd Behavior Network
Size: 5.8 MB
Format: Unknown
Cite this master thesis
APA
Lievens, F. (2022). Master thesis : Large Scale and Real-Time Cattle Behaviour Recognition by Deep Learning on Video Data. (Unpublished master's thesis). Université de Liège, Liège, Belgique. Retrieved from https://matheo.uliege.be/handle/2268.2/14427
Chicago
The University of Liège does not guarantee the scientific quality of these students' works or the accuracy of all the information they contain.