Master thesis : Evaluation of CT scan-based tumor meshing techniques and building of statistical and machine learning models to predict specific medical outcomes from mesh-based radiomic features
Lione, Maxime
Promotor(s) :
Sacré, Pierre
Date of defense : 27-Jun-2022/28-Jun-2022 • Permalink : http://hdl.handle.net/2268.2/14527
Details
Title : | Master thesis : Evaluation of CT scan-based tumor meshing techniques and building of statistical and machine learning models to predict specific medical outcomes from mesh-based radiomic features |
Translated title : | [fr] Evalutation de technique de génération de maillages à partir de scan CT et construction de modèles de machine learning basés sur des nouveaux radiomic features pour la prédiction d'outcome spécifiques |
Author : | Lione, Maxime ![]() |
Date of defense : | 27-Jun-2022/28-Jun-2022 |
Advisor(s) : | Sacré, Pierre ![]() |
Committee's member(s) : | Phillips, Christophe ![]() Geuzaine, Christophe ![]() Vaidyanathan, Akshayaa |
Language : | English |
Number of pages : | 69 |
Keywords : | [en] Artificial Intelligence [en] Tumors [en] Machine Learning [en] Radiomics [en] Medical Imaging |
Discipline(s) : | Life sciences > Biotechnology |
Research unit : | Radiomics |
Target public : | Researchers Professionals of domain Student General public |
Institution(s) : | Université de Liège, Liège, Belgique Radiomics, Liège, Belgique |
Degree: | Master en ingénieur civil biomédical, à finalité spécialisée |
Faculty: | Master thesis of the Faculté des Sciences appliquées |
Abstract
[en] This master’s thesis was conducted in conjunction with an internship at Radiomics, a next generation imaging research organization based in Liège, Belgium. The company is focusing on A.I. powered healthcare, with a unique expertise in radiomics, deep learning and federated learning applied to oncology and other therapeutic areas.
One of the main focuses of the research and development team of Radiomics is the discovery
of new pathophysiologically relevant features. Recently, new features, called the mesh-based features, which computation is based on a three-dimensional surface mesh of the tumor, have been designed by the team. By considering this type of features, we believe that we can add valuable and accurate information about the tumor surface geometry to the information provided by the already existing and well known radiomic features.
On one hand, the first objective of this work is to make use of these well-known radiomic
features to predict hepatocellular carcinoma (HCC) recurrence. This work firstly describes the
machine learning based model that has been elaborated with the radiomic features for this objective. Results revealed better predicting performance when the model was using the radiomic
features compared to when it was fed only with clinical features.
On the other hand, the other objectives relate to the new mesh-based features. The second
objective is indeed to assess the precision of different mesh generation techniques, despite the limitations on medical images accuracy that prevent the exact tumor surface geometry to be known.
Then, the third objective is to explore the relevance and predictive power of the new mesh-based
features for other specific outcomes.
Therefore, this work also describes the methodology developed to compare the precision of
different mesh generation techniques for tumor meshing from medical images. This methodology
is divided into two different approaches. In the first approach, a scan of a 3D printed cylindrical
phantom that mimics tumor tissue is used to generate meshes through different techniques, and
those meshes are compared to the ground truth phantom geometry. In the second approach, artificial images of tumors are used, in which partial volume effect and artifacts-induced blurring have been inserted. Then, a model for predicting recurrence in non-small cell lung cancers (NSCLC) with the new mesh-based features is built and a survival analysis of the new mesh-based features is driven, along with a volume dependency correction.
Both approaches to compare mesh generation techniques agreed on a given technique which
should be the most precise one and should be the one used for mesh-based features computation.
Then, the machine learning based model for predicting NSCLC recurrence revealed better predicting performance when using the new mesh-based features compared to when it was only using with clinical features. Finally, the conducted survival analysis in NSCLC showed no significant association between the new mesh-based features and the survival time, when they were corrected for their volume-dependency.
File(s)
Document(s)
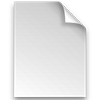

Description: Thesis
Size: 22.97 MB
Format: Adobe PDF
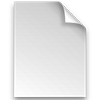

Description: abstract
Size: 65.97 kB
Format: Adobe PDF
Annexe(s)
Cite this master thesis
The University of Liège does not guarantee the scientific quality of these students' works or the accuracy of all the information they contain.