Master thesis : Predicting sudden infant death syndrome with time series based ML algorithms
Amar, Salah
Promoteur(s) :
Louppe, Gilles
;
Kleyntssens, Thomas
Date de soutenance : 5-sep-2022/6-sep-2022 • URL permanente : http://hdl.handle.net/2268.2/16286
Détails
Titre : | Master thesis : Predicting sudden infant death syndrome with time series based ML algorithms |
Titre traduit : | [fr] Prédire le syndrome de mort subite du nourrisson à l'aide d'algorithmes ML basés sur des séries temporelles |
Auteur : | Amar, Salah ![]() |
Date de soutenance : | 5-sep-2022/6-sep-2022 |
Promoteur(s) : | Louppe, Gilles ![]() Kleyntssens, Thomas |
Membre(s) du jury : | Geurts, Pierre ![]() Louveaux, Quentin ![]() |
Langue : | Anglais |
Nombre de pages : | 60 |
Mots-clés : | [fr] Sudden Infant Death Syndrom [fr] Forecasting [fr] Machine learning [fr] Time series |
Discipline(s) : | Ingénierie, informatique & technologie > Sciences informatiques |
Organisme(s) subsidiant(s) : | Comunicare Solutions |
Public cible : | Chercheurs Professionnels du domaine Etudiants |
Institution(s) : | Université de Liège, Liège, Belgique |
Diplôme : | Master en ingénieur civil en informatique, à finalité spécialisée en "intelligent systems" |
Faculté : | Mémoires de la Faculté des Sciences appliquées |
Résumé
[fr] In 2020, in the US, for every 10,000 babies 2.9 died from Sudden Infant Death Syndrome (SIDS).
SIDS is the unexpected death of infants younger than 1 year old. This phenomenon usually happens during sleep and is the leading cause of infant mortality in western countries. Sudden Infant Death Syndrome deaths are inexplicable even after a complete autopsy and analysis of the circumstances of death.
This thesis, promoted by Comunicare Solutions, aims at providing a proof of concept for the task of forecasting crises in infants with the help of a time series based machine learning algorithms. The ultimate goal of the project would be to deploy those algorithms to provide reliable and meaningful support for parents. The machine learning processes will be based on the MIMIC-III database which holds irregularly sampled time series data monitoring health signals of 7874 newborns in ICU.
The forecasting task was formulated as a sequence to sequence multi-output classification and was approached through the following main steps, a data analysis, the solving of the machine learning problem then the best model was studied further through model interpretation tasks.
The data analysis on the MIMIC-III database provided meaningful information about infants and the best time step duration to consider for the sequences. The forecasting task was solved at first for a 30 minutes time horizon with several algorithms. This led to great performances of one particular algorithm which was used in a time horizon generalization that demonstrated the temporal limit of the solution. The model interpretation tasks were split in two ways. The first one was a feature selection which aimed at reducing the number of sensors needed for the forecasting and analysing the model. Two methods were used and led to good performances with fewer features. And finally, the second way was a study of model explanation, with again two main methods, to provide some insight into the model's decisions.
Fichier(s)
Document(s)
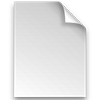

Description:
Taille: 1.24 MB
Format: Adobe PDF
Annexe(s)
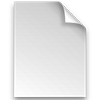

Description:
Taille: 62.73 kB
Format: Adobe PDF
Citer ce mémoire
APA
Amar, S. (2022). Master thesis : Predicting sudden infant death syndrome with time series based ML algorithms. (Unpublished master's thesis). Université de Liège, Liège, Belgique. Retrieved from https://matheo.uliege.be/handle/2268.2/16286
Chicago
L'Université de Liège ne garantit pas la qualité scientifique de ces travaux d'étudiants ni l'exactitude de l'ensemble des informations qu'ils contiennent.