Application of DeepLearning Algorithm on Minecraft
Van de Goor, Elodie
Promoteur(s) :
Ernst, Damien
Date de soutenance : 8-sep-2016/9-sep-2016 • URL permanente : http://hdl.handle.net/2268.2/1686
Détails
Titre : | Application of DeepLearning Algorithm on Minecraft |
Titre traduit : | [fr] Application d'une méthode d'apprentissage profond dans Minecraft |
Auteur : | Van de Goor, Elodie ![]() |
Date de soutenance : | 8-sep-2016/9-sep-2016 |
Promoteur(s) : | Ernst, Damien ![]() |
Membre(s) du jury : | Geurts, Pierre ![]() Wehenkel, Louis ![]() Gemine, Quentin ![]() François-Lavet, Vincent ![]() |
Langue : | Anglais |
Nombre de pages : | 73 |
Mots-clés : | [en] deep learning [en] Machine learning [en] Minecraft [en] Deer [en] DeepMind [en] deep Q-network [en] reinforcement learning |
Discipline(s) : | Ingénierie, informatique & technologie > Sciences informatiques |
Public cible : | Chercheurs Professionnels du domaine Etudiants |
Institution(s) : | Université de Liège, Liège, Belgique |
Diplôme : | Master en ingénieur civil en informatique, à finalité approfondie |
Faculté : | Mémoires de la Faculté des Sciences appliquées |
Résumé
[en] Some years ago, Google DeepMind released a paper describing an agent architecture, DQN. This agent was able to learn to play better than humans in 49 different Atari game while receiving only the game screen and scores as inputs. With these kinds of results we can ask ourselves how well this agent could do in the environment of a new game. The purpose of this thesis is to make the DeepMind agent evolve into Minecraft and make it easily adaptable to many kinds of tasks. Its task is to destroy as many mobs as possible in a room.
The implementation of a DeepMind agent has been carried out in the game Minecraft through the ULg program Deer. The communication between Deer and minecraft was designed in a modular way so it can be adapted to other tasks or even other games. A number of experiments have been conducted to test different combination of parameters.
The learning speed of the agent was impressive when we consider the small learning phase it has comparing to the Atari learning phases. It made good results and when we increase the number of step to 80,000 it was as good as a human player, even developing strategies to find and trap the mobs. However it still has a stability problem.
These results are encouraging and more tests should help to reach an even better score. Once this is done, the difficulty of the task can be increased to a moving agent for example. Step by step, it is possible to test more and more complex tasks by making the agent evolve with other paper results or other machine learning mechanisms. This environment will allow making both the agents architecture and the tasks evolve to whatever one would want.
Fichier(s)
Document(s)
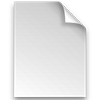

Description:
Taille: 9.42 MB
Format: Adobe PDF
Annexe(s)
Citer ce mémoire
L'Université de Liège ne garantit pas la qualité scientifique de ces travaux d'étudiants ni l'exactitude de l'ensemble des informations qu'ils contiennent.