Novel viewpoint synthesis of sport scenes using broadcast images
Birtles, David
Promoteur(s) :
Louppe, Gilles
;
Hoyoux, Thomas
Date de soutenance : 26-jui-2023/27-jui-2023 • URL permanente : http://hdl.handle.net/2268.2/17391
Détails
Titre : | Novel viewpoint synthesis of sport scenes using broadcast images |
Auteur : | Birtles, David ![]() |
Date de soutenance : | 26-jui-2023/27-jui-2023 |
Promoteur(s) : | Louppe, Gilles ![]() Hoyoux, Thomas |
Membre(s) du jury : | Van Droogenbroeck, Marc ![]() Geurts, Pierre ![]() Hoyoux, Thomas |
Langue : | Anglais |
Nombre de pages : | 67 |
Mots-clés : | [en] Deep learning [en] NeRF [en] Machine learning [en] Computer Vision [fr] Apprentissage profond [fr] Apprentissage automatique [fr] NeRF [fr] Vision par ordinateur |
Discipline(s) : | Ingénierie, informatique & technologie > Sciences informatiques |
Institution(s) : | Université de Liège, Liège, Belgique |
Diplôme : | Master en ingénieur civil en informatique, à finalité spécialisée en "intelligent systems" |
Faculté : | Mémoires de la Faculté des Sciences appliquées |
Résumé
[en] NeRF is a recent method for novel view synthesis and proved its capabilities by enabling the rendering of truly photorealistic novel views of a scene, only leveraging calibrated images. This method is able to render high-quality images when trained with many views densely distributed in translation and rotation around the scene. However, its performances degrade when used in sport broadcasting conditions where the number of cameras is limited and are only able to move in rotation. Furthermore, the original NeRF is designed to work on static scenes and is very slow both for training and inference. Both of these factor limits the application of NeRF in sport broadcasting conditions, where moving elements are abundant and live delivery is required. In this work, we only touch upon the problem of time constraints and leave aside the problem of moving elements. Instead, we focus on the problem of sparse input views of a static scene: we analyse and quantify how the performances of NeRF are limited by the number of viewpoints in the training set. We show that using depth information combined with a depth loss greatly improves results even if we only have partial depth information. We integrate this extension with the nerfacto model, which is an off-the-shelf NeRF model several orders of magnitude faster to train and to render images than the original NeRF. Furthermore, we implement and integrate with nerfacto a patch-based regularization technique, also meant to alleviate the problem of sparse input views. While the latter extension does not bring the expected performance improvement, the resulting model is overall much faster than the original NeRF while providing greatly improved results in a sparse input view setup characteristic of sport broadcasting conditions.
Fichier(s)
Document(s)
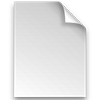

Description:
Taille: 89.38 MB
Format: Adobe PDF
Citer ce mémoire
APA
Birtles, D. (2023). Novel viewpoint synthesis of sport scenes using broadcast images. (Unpublished master's thesis). Université de Liège, Liège, Belgique. Retrieved from https://matheo.uliege.be/handle/2268.2/17391
Chicago
L'Université de Liège ne garantit pas la qualité scientifique de ces travaux d'étudiants ni l'exactitude de l'ensemble des informations qu'ils contiennent.