Master Thesis : Deblurring of Sports Videos using Deep Learning
Delcour, Florian
Promotor(s) :
Louppe, Gilles
Date of defense : 26-Jun-2023/27-Jun-2023 • Permalink : http://hdl.handle.net/2268.2/17702
Details
Title : | Master Thesis : Deblurring of Sports Videos using Deep Learning |
Translated title : | [fr] Réduction du flou de vidéos sportives par apprentissage profond |
Author : | Delcour, Florian ![]() |
Date of defense : | 26-Jun-2023/27-Jun-2023 |
Advisor(s) : | Louppe, Gilles ![]() |
Committee's member(s) : | Cioppa, Anthony ![]() Marée, Raphaël ![]() Castin, Martin |
Language : | English |
Number of pages : | 78 |
Keywords : | [en] deep-learning [en] deblurring [en] EVS Broadcast [en] sports [en] videos [en] fine-tuning [en] slow-motion |
Discipline(s) : | Engineering, computing & technology > Computer science |
Target public : | Researchers Professionals of domain |
Institution(s) : | Université de Liège, Liège, Belgique |
Degree: | Master : ingénieur civil en science des données, à finalité spécialisée |
Faculty: | Master thesis of the Faculté des Sciences appliquées |
Abstract
[en] Nowadays, sports videos are among the most consumed forms of entertainment and viewers expect visually appealing and smooth content. Slow-motion replay has become an essential feature in sports broadcasting but typically requires expensive high-technology hardware.
To mitigate these costs, an alternative approach is to interpolate frames from low-frequency cameras to simulate slow-motion. However, motion blur, naturally present in sports videos at normal speed, becomes increasingly disturbing as the playback speed decreases. In this context, video deblurring aims to restore sharpness in blurry videos, using the correlated information present in consecutive frames. In this thesis, we first compare various deep video deblurring methods on commonly used datasets and on real sports videos. Afterwards, we generate our own sports synthetic dataset, which consists of pairs of blurry-sharp frame sequences. The blurry sequences are obtained by averaging consecutive frames of sports videos to simulate motion blur. Using the generated dataset, we then fine-tune a pretrained neural network called RVRT, which showed to be the most promising model. Lastly, a series of experiments are conducted to analyze the deblurring performance of the model in several scenarios. We demonstrate that our fine-tuned model significantly outperforms the pretrained model both quantitatively and qualitatively on our generated blurry sports dataset, achieving PSNR of 35.98 (+3.65dB) and SSIM of 0.9338 (+0.0214) on the validation set. The model successfully restores sharp fine-grained details even in complex real-blur scenarios. Nonetheless, commercial applications are currently unfeasible due to the large inference time and the presence of a few remaining artifacts and blurry local regions.
File(s)
Document(s)
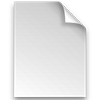

Description:
Size: 88.57 MB
Format: Adobe PDF
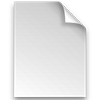

Description:
Size: 185.34 kB
Format: Adobe PDF
Annexe(s)
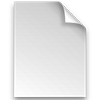

Description:
Size: 196.59 MB
Format: Unknown
Cite this master thesis
APA
Delcour, F. (2023). Master Thesis : Deblurring of Sports Videos using Deep Learning. (Unpublished master's thesis). Université de Liège, Liège, Belgique. Retrieved from https://matheo.uliege.be/handle/2268.2/17702
Chicago
The University of Liège does not guarantee the scientific quality of these students' works or the accuracy of all the information they contain.