Master Thesis : A Chatbot-Driven Search Engine for Improved Data Accessibility
Merle, Corentin
Promotor(s) :
Ittoo, Ashwin
Date of defense : 4-Sep-2023/5-Sep-2023 • Permalink : http://hdl.handle.net/2268.2/18353
Details
Title : | Master Thesis : A Chatbot-Driven Search Engine for Improved Data Accessibility |
Translated title : | [fr] Assistant de Recherche pour Améliorer l'Accessibilité des Données |
Author : | Merle, Corentin ![]() |
Date of defense : | 4-Sep-2023/5-Sep-2023 |
Advisor(s) : | Ittoo, Ashwin ![]() |
Committee's member(s) : | Huynh-Thu, Vân Anh ![]() Debruyne, Christophe ![]() Jacquerie, Jean-Louis |
Language : | English |
Number of pages : | 100 |
Keywords : | [fr] Chatbot [fr] Search Engine [fr] LLM [fr] Large Language Model [fr] Knowledge Graph |
Discipline(s) : | Engineering, computing & technology > Civil engineering |
Institution(s) : | Université de Liège, Liège, Belgique |
Degree: | Master : ingénieur civil en science des données, à finalité spécialisée |
Faculty: | Master thesis of the Faculté des Sciences appliquées |
Abstract
[fr] Increasingly, large organizations are faced with the challenge of making data accessible and understandable to non-expert users. Despite the advances in natural language processing and knowledge representation, turning data into natural language responses that can be understood by a general audience remains a significant challenge. Moreover, this issue is exacerbated by the exponential growth of information and the fragmentation of data into isolated silos, which underscores the urgent need for tools to provide more straightforward, single-point data access.
This thesis aims to address these challenges by introducing the use of Enterprise Knowledge Graphs as a unified data structure for consolidating and representing disparate data sources, coupled with SPARCoder, our ontology-aware Text-to- SPARQL fine-tuned Large Language Model based on StarCoder (Li et al. 2023), capable of querying knowledge graphs to retrieve data using natural language. The proposed natural language "search engine" architecture leverages the strengths of Large Language Models in understanding and generating human-like text, combined with the structured representation of information provided by knowledge graphs. In essence, this approach bridges the gap between complex data and end-users, offering a more accessible interface.
In this work, we undertake a comprehensive description of our proposed system, contrasting its advantages and drawbacks with traditional methods of data access and retrieval as well as other state-of-the-art large language models.
Consequently, we assert that the integration of large language models with knowledge graph querying significantly improves data accessibility for non-expert users. The proposed "search engine" prototype not only facilitates a more intuitive and accessible way of interacting with data but also opens up new possibilities for user interaction, leading to more informed and data-driven decision making.
File(s)
Document(s)
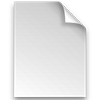

Description: -
Size: 14.91 MB
Format: Adobe PDF
Annexe(s)
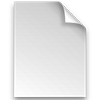

Description: -
Size: 19.27 MB
Format: Unknown
Cite this master thesis
The University of Liège does not guarantee the scientific quality of these students' works or the accuracy of all the information they contain.