Master thesis : Classification of aluminum alloy scraps via spectral analysis: A deep learning approach to laser-induced breakdown spectroscopy
Schwanen, Julien
Promoteur(s) :
Louppe, Gilles
Date de soutenance : 26-jan-2024 • URL permanente : http://hdl.handle.net/2268.2/19558
Détails
Titre : | Master thesis : Classification of aluminum alloy scraps via spectral analysis: A deep learning approach to laser-induced breakdown spectroscopy |
Auteur : | Schwanen, Julien ![]() |
Date de soutenance : | 26-jan-2024 |
Promoteur(s) : | Louppe, Gilles ![]() |
Membre(s) du jury : | Drion, Guillaume ![]() Geurts, Pierre ![]() Baudinet, Charles ![]() |
Langue : | Anglais |
Nombre de pages : | 137 |
Mots-clés : | [en] Deep Learning [en] Alloy Classification [en] Laser-Induced Breakdown Spectroscopy (LIBS) [en] Signal Processing [en] Spectral Analysis [en] Pattern Recognition [en] Aluminum Waste [en] Metal Recycling [en] Material Identification [en] Deep Neural Networks [en] Alloy Characterization [en] Spectroscopic Data Analysis |
Discipline(s) : | Ingénierie, informatique & technologie > Ingénierie civile |
Organisme(s) subsidiant(s) : | Université de Liège |
Centre(s) de recherche : | Laboratoire du GeMMe |
Public cible : | Autre |
Institution(s) : | Université de Liège, Liège, Belgique |
Diplôme : | Master en ingénieur civil en informatique, à finalité spécialisée en "intelligent systems" |
Faculté : | Mémoires de la Faculté des Sciences appliquées |
Résumé
[en] This work explores innovative methodologies in metal classification, focusing on
aluminum, within the GeMMe laboratory’s framework and the SALEMA project.
This research focuses on three main objectives: firstly, to maximize information
extraction from the complete spectral data for aluminum classification; secondly, to
deepen understanding of the set structure of the aluminum samples composed of
multiple Laser-Induced Breakdown Spectroscopy spectra; and thirdly, to enhance
classification techniques by integrating unsupervised datasets.
This work employs various deep learning models, including the Multi-Layer Percep tron (MLP), Residual Network (ResNet), Variational Autoencoder (VAE), and their
skip-connected variant. It compares them against GeMMe’s baseline in preprocessed
and non-preprocessed data scenarios.
Experimental findings suggest that a comprehensive analysis of the entire spectrum
of each Laser-Induced Breakdown Spectroscopy spectra contributes to an improved
weighted F1 of 2% compared to the GeMMe’s PICKIT baseline. Secondly, acknowl edging the set structure of the samples, further refines the classification, particularly
with the application of deep set techniques. This approach has shown a significant
increase in weighted F1 score of 11% compared to the GeMMe’s PICKIT baseline.
Additionally, the introduction of unsupervised datasets in the context of generative
models, particularly the skip-connected VAE, markedly enhanced the weighted F1
of 1.3%, surpassing the previously mentioned approach, especially for smaller sets.
This was achieved by balancing the generative and the classification loss.
The model’s generalization capabilities were tested on unseen datasets, showing
weighted F1 over 85%, yet indicating challenges in samples with fewer spectra.
Fichier(s)
Document(s)
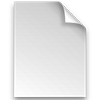

Description: -
Taille: 29.41 MB
Format: Adobe PDF
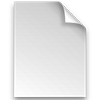

Description: -
Taille: 241.63 kB
Format: Adobe PDF
Citer ce mémoire
L'Université de Liège ne garantit pas la qualité scientifique de ces travaux d'étudiants ni l'exactitude de l'ensemble des informations qu'ils contiennent.