Super Resolution Using Diffusion Models
Alrahel, Badei
Promotor(s) :
Louppe, Gilles
Date of defense : 24-Jun-2024/25-Jun-2024 • Permalink : http://hdl.handle.net/2268.2/20475
Details
Title : | Super Resolution Using Diffusion Models |
Translated title : | [fr] Tirer parti des techniques avancées de modèle de diffusion pour la super-résolution des images de diffusion sportive |
Author : | Alrahel, Badei ![]() |
Date of defense : | 24-Jun-2024/25-Jun-2024 |
Advisor(s) : | Louppe, Gilles ![]() |
Committee's member(s) : | Botta, Vincent
Geurts, Pierre ![]() Huynh-Thu, Vân Anh ![]() |
Language : | English |
Number of pages : | 86 |
Keywords : | [fr] Diffusion models [fr] Super-Resolution |
Discipline(s) : | Engineering, computing & technology > Computer science |
Institution(s) : | Université de Liège, Liège, Belgique |
Degree: | Master en science des données, à finalité spécialisée |
Faculty: | Master thesis of the Faculté des Sciences appliquées |
Abstract
[fr] This thesis investigates the application of diffusion models to the task of image super-resolution,
a crucial process for enhancing the quality of low-resolution images. Diffusion models, known
for their success in generative tasks, are examined for their potential to outperform traditional
super-resolution methods. The study begins with an overview of generative models, including
Variational Autoencoders (VAEs) and their hierarchical variants, and introduces the core principles
of diffusion models, detailing key processes such as forward diffusion, reverse denoising, and
their three equivalent interpretations.
The literature review covers both regression-based and generative-based super-resolution methods,
highlighting the strengths and limitations of techniques like Generative Adversarial Networks
(GANs) and various diffusion-based models. Notably, models such as SR3 (Super-Resolution via
Iterative Refinement) and Latent Diffusion Models (LDMs) are examined for their efficiency and
performance.
A proof-of-concept implementation of the SR3 model is conducted to demonstrate the practical
application and effectiveness of diffusion models in super-resolution tasks, particularly when
compared to the regression-based method A
2N, with a focus on reconstructing facial images.
The thesis introduces and compares advanced models, Efficient Diffusion Model for Image
Super-resolution by Residual Shifting (ResShift) and Diffusion-Based Image Super-Resolution
in a Single Step (SinSR), which offer significant improvements in inference speed and image
quality. Specifically, an implementation of SinSR on top of ResShift is proposed.
An effective data pipeline utilizing blind super-resolution techniques, along with random cropping
and resizing, is proposed for training the implemented SinSR model. This pipeline is compared
to a simpler cropping-only approach showing the importance of the training data scale that are
given to the model as input.
Extensive experiments were conducted to evaluate the performance of the proposed methods,
focusing particularly on sports broadcast and facial image data. The evaluation includes an
assessment of the performance of the used VQGAN within the SinSR model, an experiment
evaluating the model’s performance relative to varying input face sizes, and a study comparing
the training of the model on blurry versus non-blurry images.
File(s)
Document(s)
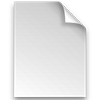

Description: -
Size: 71.78 MB
Format: Adobe PDF
Cite this master thesis
The University of Liège does not guarantee the scientific quality of these students' works or the accuracy of all the information they contain.