Efficient Image Pre-Training with Siamese Cropped Masked Autoencoders
Eymaël, Alexandre
Promotor(s) :
Van Droogenbroeck, Marc
Date of defense : 24-Jun-2024/25-Jun-2024 • Permalink : http://hdl.handle.net/2268.2/20476
Details
Title : | Efficient Image Pre-Training with Siamese Cropped Masked Autoencoders |
Author : | Eymaël, Alexandre ![]() |
Date of defense : | 24-Jun-2024/25-Jun-2024 |
Advisor(s) : | Van Droogenbroeck, Marc ![]() |
Committee's member(s) : | Cioppa, Anthony ![]() Geurts, Pierre ![]() |
Language : | English |
Number of pages : | 102 |
Keywords : | [en] Machine Learning [en] Deep Learning [en] Computer Vision [en] Self-Supervised Learning [en] Masked Autoencoders [en] Siamese Networks [en] Video Segmentation [en] Label Propagation |
Discipline(s) : | Engineering, computing & technology > Computer science |
Commentary : | A paper related to this master's thesis, of which I am the first author, was submitted to the main conference of the European Conference on Computer Vision (ECCV) 2024. The paper is available on arXiv at the following link: https://arxiv.org/abs/2403.17823. |
Research unit : | Telecommunications and Imaging Laboratory, Institut Montefiore, Université de Liège |
Target public : | Researchers Professionals of domain Student |
Institution(s) : | Université de Liège, Liège, Belgique |
Degree: | Master en science des données, à finalité spécialisée |
Faculty: | Master thesis of the Faculté des Sciences appliquées |
Abstract
[en] Self-supervised pre-training of image encoders has become omnipresent in the literature, especially since the introduction of Masked Autoencoders (MAE). To excel in propagation tasks such as video segmentation, current research focuses on learning object-centric representations from video motion. Notably, SiamMAE introduced a Siamese network that trains a shared-weight encoder from two video frames with a high asymmetric masking ratio (95%), achieving state-of-the-art performance in video object segmentation, human pose propagation, and semantic part propagation.
In this work, we propose CropMAE, an alternative to the Siamese pre-training method introduced by SiamMAE. Unlike SiamMAE that uses pairs of frames from videos, CropMAE exclusively considers pairs of cropped images sourced from the same still image, but cropped differently. This approach eliminates the need for video decoding, enabling training on still image datasets and significantly reducing pre-training time while maintaining competitive performance.
Our empirical results demonstrate that CropMAE can learn object-centric representations without relying on motion, unlike SiamMAE. This discovery indicates that with the appropriate pretext task, it is possible to acquire object-centric features without using videos or motion information. Furthermore, we show that the pretext task in CropMAE is more explicit and accelerates the learning process of object-centric representations compared to SiamMAE. Additionally, CropMAE achieves the highest masking ratio to date (98.5%), allowing image reconstruction with only two visible patches.
File(s)
Document(s)
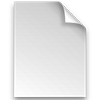

Description: Abstract
Size: 47.38 kB
Format: Adobe PDF
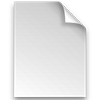

Description: Thesis
Size: 56.88 MB
Format: Adobe PDF
Cite this master thesis
The University of Liège does not guarantee the scientific quality of these students' works or the accuracy of all the information they contain.