Predicting Production and Assembly Times in Steel Manufacturing Using Machine Learning
Meunier, Loïc
Promoteur(s) :
Geurts, Pierre
Date de soutenance : 5-sep-2024/6-sep-2024 • URL permanente : http://hdl.handle.net/2268.2/21031
Détails
Titre : | Predicting Production and Assembly Times in Steel Manufacturing Using Machine Learning |
Auteur : | Meunier, Loïc ![]() |
Date de soutenance : | 5-sep-2024/6-sep-2024 |
Promoteur(s) : | Geurts, Pierre ![]() |
Membre(s) du jury : | Wehenkel, Louis ![]() Drion, Guillaume ![]() |
Langue : | Anglais |
Discipline(s) : | Ingénierie, informatique & technologie > Sciences informatiques |
Commentaire : | This thesis was conducted in collaboration with the belgian company VVTW (see https://vvtw.be/). |
Institution(s) : | Université de Liège, Liège, Belgique |
Diplôme : | Master en sciences informatiques, à finalité spécialisée en "computer systems security" |
Faculté : | Mémoires de la Faculté des Sciences appliquées |
Résumé
[en] The steel manufacturing industry is undergoing a gradual transition towards the integration of digital processes throughout its entire supply chain. New technologies, such as artificial intelligence, have been the subject of greater exposure, and new possibilities have been identified for enhancing production planning.
In collaboration with the VVTW company, this study seeks to develop data-driven models for the precise prediction of production and assembly times for a range of steel components, including positions and assemblies.
The initial step involved the extraction of meaningful data from the company’s comprehensive database. Following a thorough examination of the data and the extraction of valuable insights for the company, three distinct models were refined and evaluated in comparison to a representative baseline currently employed in steel factories.
With regard to the evaluation of positions, the Random Forest model exhibited the most favorable performance, demonstrating a 71.05% and 74.93% improvement of RMSE over the baseline, respectively, for plates and profiles. Concerning assemblies, the most effective model was also the Random Forest, which yielded an improvement of 60.04% in RMSPE relative to the baseline. Additionally, a particular category of low-weight assemblies appeared to be more challenging to accurately predict. To address this, two potential explanations were put forth.
Ultimately, the data-driven models were incorporated into the company’s software platform for implementation with other clientele.
Fichier(s)
Document(s)
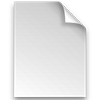

Description: Full thesis, including appendices.
Taille: 5.62 MB
Format: Adobe PDF
Citer ce mémoire
L'Université de Liège ne garantit pas la qualité scientifique de ces travaux d'étudiants ni l'exactitude de l'ensemble des informations qu'ils contiennent.