Master's Thesis : Data modelling of steam turbine performance
Lamborelle, Maxime
Promoteur(s) :
Wehenkel, Louis
Date de soutenance : 25-jui-2020/26-jui-2020 • URL permanente : http://hdl.handle.net/2268.2/9022
Détails
Titre : | Master's Thesis : Data modelling of steam turbine performance |
Auteur : | Lamborelle, Maxime ![]() |
Date de soutenance : | 25-jui-2020/26-jui-2020 |
Promoteur(s) : | Wehenkel, Louis ![]() |
Membre(s) du jury : | Geurts, Pierre ![]() Louppe, Gilles ![]() Demarneffe, Renaud |
Langue : | Anglais |
Mots-clés : | [fr] Anomaly Detection [fr] Steam Turbine [fr] Condition Based Maintenance |
Discipline(s) : | Ingénierie, informatique & technologie > Sciences informatiques |
Institution(s) : | Université de Liège, Liège, Belgique |
Diplôme : | Master : ingénieur civil en science des données, à finalité spécialisée |
Faculté : | Mémoires de la Faculté des Sciences appliquées |
Résumé
[fr] Maintaining industrial steam turbines is a very difficult task. It is now possible, using data- driven methods, to detect anomalies quickly to be able to fix them before the anomalies get bigger. In this project, a systematic methodology to detect anomalies on steam turbines is developed. Taking data coming from sensors placed in the turbine we can output the main anomalies as well as the main possible causes of the anomalies. Different algorithms are at the core of the anomaly detection process. One of them, based on Recurrent Neural Network, is a new architecture that can detect big anomalies as well as smaller anomalies. This methodology was developed on a first turbine and then applied to a different turbine proving that this newly developed methodology is systematic and only needs a few days to be adapted to a different case. The ability of this approach to successfully detect steam turbine anomalies and identify the most probable causes of these anomalies has been validated by experts.
Fichier(s)
Document(s)
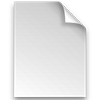

Description:
Taille: 14.47 MB
Format: Adobe PDF
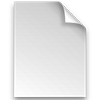

Description: One page Summary
Taille: 80.72 kB
Format: Adobe PDF
Annexe(s)
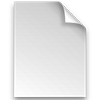

Description: Python scripts and notebooks
Taille: 7.64 MB
Format: Unknown
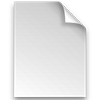

Description: Representative illustrations
Taille: 860.88 kB
Format: Unknown
Citer ce mémoire
L'Université de Liège ne garantit pas la qualité scientifique de ces travaux d'étudiants ni l'exactitude de l'ensemble des informations qu'ils contiennent.