Master's Thesis : Semi-supervised learning for object detection in satellite images
Vandeghen, Renaud
Promotor(s) :
Louppe, Gilles
Date of defense : 25-Jun-2020/26-Jun-2020 • Permalink : http://hdl.handle.net/2268.2/9053
Details
Title : | Master's Thesis : Semi-supervised learning for object detection in satellite images |
Translated title : | [fr] Apprentissage semi-supervisé pour la detection d'objets dans des images satellites |
Author : | Vandeghen, Renaud ![]() |
Date of defense : | 25-Jun-2020/26-Jun-2020 |
Advisor(s) : | Louppe, Gilles ![]() |
Committee's member(s) : | Van Droogenbroeck, Marc ![]() Wehenkel, Louis ![]() Denies, Jonathan |
Language : | English |
Number of pages : | 51 |
Keywords : | [fr] deep learning [fr] semi-supervised learning [fr] object detection [fr] remote sensing |
Discipline(s) : | Engineering, computing & technology > Electrical & electronics engineering |
Funders : | Aerospacelab |
Target public : | Researchers Professionals of domain Student |
Institution(s) : | Université de Liège, Liège, Belgique |
Degree: | Master : ingénieur civil électricien, à finalité spécialisée en "signal processing and intelligent robotics" |
Faculty: | Master thesis of the Faculté des Sciences appliquées |
Abstract
[en] Geospatial intelligence is growing very fast trough deep learning models. Unfortunately those models often rely on large labeled dataset, which are expensive to build. An interesting solution to improve the performance without manually annotating more data is to use semi- supervised learning methods, which have shown promising potential to leverage unlabeled data.
Very good improvement with semi-supervised learning has already
be done in the context of image classification, although very few works have been conducted for object detection.
This master thesis aim to implement and study different semi-supervised learning methods for object detection in satellite images.
Eventually, two semi-supervised learning methods are proposed, namely NSTOD and CODDA.
NSTOD is a self-training method which adds noise during the train- ing and then produces pseudo-labels to enlarge the training dataset. CODDA is a consistency based method which forces the network to have consistent prediction when data augmentation is applied.
The performance is evaluated on the twenty classes of DIOR and on a single object class to assess this performance for geospatial intelli- gence application.
On a single class object focusing on storage tanks, NSTOD improves from 71.3% to 81.7% the AP while considering the same labeled dataset composed of 85 images.
File(s)
Document(s)
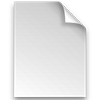

Description:
Size: 92.33 kB
Format: Adobe PDF
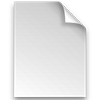

Description:
Size: 77.94 MB
Format: Adobe PDF
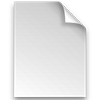

Description: -
Size: 92.31 kB
Format: Adobe PDF
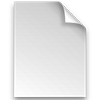

Description: -
Size: 77.94 MB
Format: Adobe PDF
Annexe(s)
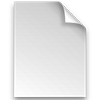

Description:
Size: 86.7 kB
Format: Unknown
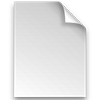

Description:
Size: 1.54 MB
Format: image/png
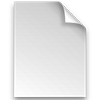

Description:
Size: 946.97 kB
Format: image/png
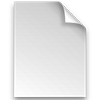

Description:
Size: 1.51 MB
Format: image/png
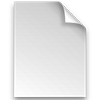

Description:
Size: 923.84 kB
Format: image/png
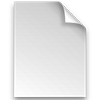

Description:
Size: 1.68 MB
Format: image/png
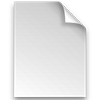

Description:
Size: 2.68 MB
Format: image/png
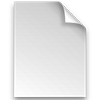

Description:
Size: 2.85 MB
Format: image/png
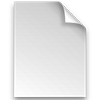

Description:
Size: 1.62 MB
Format: image/png
Cite this master thesis
The University of Liège does not guarantee the scientific quality of these students' works or the accuracy of all the information they contain.