Modeling systemic risk in the banking industry via a network of extremal dependencies - An approach based on Generalized Pareto regression and the LASSO
Nikkels, Mirko
Promoteur(s) :
Hambuckers, Julien
Date de soutenance : 2-sep-2020/8-sep-2020 • URL permanente : http://hdl.handle.net/2268.2/10190
Détails
Titre : | Modeling systemic risk in the banking industry via a network of extremal dependencies - An approach based on Generalized Pareto regression and the LASSO |
Titre traduit : | [fr] Modélisation du risque systémique au sein de l'industrie bancaire via un réseau de dépendances d'extrema. Une approche basée sur une régression de Pareto généralisée et le LASSO. |
Auteur : | Nikkels, Mirko ![]() |
Date de soutenance : | 2-sep-2020/8-sep-2020 |
Promoteur(s) : | Hambuckers, Julien ![]() |
Membre(s) du jury : | Ittoo, Ashwin ![]() Moreno Miranda, Nicolas ![]() |
Langue : | Anglais |
Nombre de pages : | 82 |
Mots-clés : | [en] Systemic risk [en] Generalized Pareto Distribution [en] GPD [en] Generalized Pareto Regression [en] GPR [en] Least Absolute Shrinkage and Selection Operator [en] LASSO [en] Extreme events [en] Banking network [en] Extremal dependencies [en] Systemic risk measure [en] Value-at-Risk [en] VaR [en] EVT [en] Extreme Value Theory [en] POT [en] Peaks-Over-Thresholds [en] Banking industry [fr] Risque systémique [fr] Extrema [fr] Secteur bancaire [fr] LASSO [fr] Pareto généralisée |
Discipline(s) : | Sciences économiques & de gestion > Finance Sciences économiques & de gestion > Economie internationale Sciences économiques & de gestion > Méthodes quantitatives en économie & gestion |
Public cible : | Chercheurs Professionnels du domaine Etudiants |
Institution(s) : | Université de Liège, Liège, Belgique |
Diplôme : | Master en ingénieur de gestion, à finalité spécialisée en Financial Engineering |
Faculté : | Mémoires de la HEC-Ecole de gestion de l'Université de Liège |
Résumé
[en] In this master thesis, we investigate the interdependency among 26 global banks, using the daily closings. We especially focus on extreme negative results and, therefore, use a certain threshold to only retain exceedances from the original data. The aim is to find, for each bank from the sample, the most significant subset of covariates explaining its daily returns thanks to past returns. To do so, the proposed methodology is based on Generalized Pareto Regressions and the Least Absolute Shrinkage and Selection Operator (LASSO). If the threshold is selected sufficiently high, we can consider our analysis to be part of the Extreme Value Theory and we can apply Peaks-Over-Thresholds methods. Consequently, it is possible to model the exceedances by a Generalized Pareto Distribution with scale and shape parameters. The selection of significant covariates is performed by the penalizing technique LASSO. Only significant regression coefficients are strong enough to not be shrunk exactly to zero by the penalty. While both of the time-varying parameters are functions of the covariates, the shape parameter will be focused on drawing conclusions.
We are truly convinced that the result of this work could be used to create an additional systemic risk measure. This should especially target banks exhibiting asset levels above $1000B. and banks originating from Europe, as these banks are more exposed and interconnected.
Fichier(s)
Document(s)
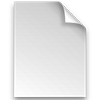

Description:
Taille: 1.88 MB
Format: Adobe PDF
Citer ce mémoire
APA
Nikkels, M. (2020). Modeling systemic risk in the banking industry via a network of extremal dependencies - An approach based on Generalized Pareto regression and the LASSO. (Unpublished master's thesis). Université de Liège, Liège, Belgique. Retrieved from https://matheo.uliege.be/handle/2268.2/10190
Chicago
L'Université de Liège ne garantit pas la qualité scientifique de ces travaux d'étudiants ni l'exactitude de l'ensemble des informations qu'ils contiennent.