Master's Thesis : Comparison of probabilistic forecasting deep learning models in the context of renewable energy production
Stassen, Théo
Promoteur(s) :
Ernst, Damien
Date de soutenance : 7-sep-2020/9-sep-2020 • URL permanente : http://hdl.handle.net/2268.2/10466
Détails
Titre : | Master's Thesis : Comparison of probabilistic forecasting deep learning models in the context of renewable energy production |
Titre traduit : | [fr] Comparison de modèles de prédiction probabilistique utilisant l'apprentissage profond dans le contexte de la production d'énergie renouvelable |
Auteur : | Stassen, Théo ![]() |
Date de soutenance : | 7-sep-2020/9-sep-2020 |
Promoteur(s) : | Ernst, Damien ![]() |
Membre(s) du jury : | Gemine, Quentin ![]() Mathieu, Sébastien ![]() |
Langue : | Anglais |
Nombre de pages : | 99 |
Mots-clés : | [en] artificial intelligence [en] machine learning [en] forecasting [en] time series forecasting [en] probabilistic forecasting [en] deep learning [en] forecasting models [en] renewable energy [en] renewable energy production [en] model comparison |
Discipline(s) : | Ingénierie, informatique & technologie > Sciences informatiques |
Public cible : | Chercheurs Professionnels du domaine Etudiants Grand public |
Institution(s) : | Université de Liège, Liège, Belgique |
Diplôme : | Master en ingénieur civil en informatique, à finalité spécialisée en "intelligent systems" |
Faculté : | Mémoires de la Faculté des Sciences appliquées |
Résumé
[en] This master thesis subject addresses the question of what is the best forecasting method to implement in the context of the prediction of renewable energy production, to protect assets from oversupply. The growing scientific field of Deep Learning has a great potential to be exploited to achieve this goal. This works is composed of different parts. First part introduces the goal that we want to be pursued. The second part interrogates what what are the tools needed to accomplish the goal and defines the context on which the comparison will be performed. The third part is a comparison of the models considering a default forecasting goal . The fourth part is a discussion on what might be the most relevant metric considering the main goal. From this we define two metrics, Coverage and MASE and we finally perform in fifth part a comparison using metrics and loss that have been introduced .
The answer to the question of what is the better forecasting model in the defined context between all the tested models, the model that provides the better results, in terms of Coverage and MASE, is definitely the model MQCNN, which outperforms for the two metrics considered all the other presented models. MQCNN model is followed by MQRNN, DeepAr and SimpleFeedForward.
Fichier(s)
Document(s)
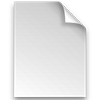

Description: Master Thesis Summary
Taille: 102.19 kB
Format: Adobe PDF
Citer ce mémoire
L'Université de Liège ne garantit pas la qualité scientifique de ces travaux d'étudiants ni l'exactitude de l'ensemble des informations qu'ils contiennent.