Master's Thesis : Lightning Gravitational Wave Parameter Inference through Neural Amortization
Delaunoy, Arnaud
Promoteur(s) :
Louppe, Gilles
Date de soutenance : 7-sep-2020/9-sep-2020 • URL permanente : http://hdl.handle.net/2268.2/10554
Détails
Titre : | Master's Thesis : Lightning Gravitational Wave Parameter Inference through Neural Amortization |
Auteur : | Delaunoy, Arnaud ![]() |
Date de soutenance : | 7-sep-2020/9-sep-2020 |
Promoteur(s) : | Louppe, Gilles ![]() |
Membre(s) du jury : | Geurts, Pierre ![]() Wehenkel, Louis ![]() Hermans, Joeri ![]() |
Langue : | Anglais |
Nombre de pages : | 63 |
Mots-clés : | [en] Gravitational wave [en] Deep learning [en] Machine learning [en] Simulation-based inference [en] Fast inference |
Discipline(s) : | Ingénierie, informatique & technologie > Sciences informatiques Physique, chimie, mathématiques & sciences de la terre > Physique |
Centre(s) de recherche : | Montefiore Institute, University of Liège GRAPPA Institute, University of Amsterdam |
Intitulé du projet de recherche : | Lightning Gravitational Wave Parameter Inference through Neural Amortization |
Public cible : | Chercheurs Professionnels du domaine |
Institution(s) : | Université de Liège, Liège, Belgique |
Diplôme : | Master : ingénieur civil en science des données, à finalité spécialisée |
Faculté : | Mémoires de la Faculté des Sciences appliquées |
Résumé
[en] Gravitational waves analysis relies on a simulator governed by the nonlinear field equations of general relativity for binary systems. Such analysis is computationally very expensive and necessitates a large-scale exploration of the likelihood surface over the full parameter space.
Neural networks have been gaining popularity as tools for gravitational waves analysis for the last few years. They lead to fast gravitational wave detection and parameter inference and hence complement classical slower techniques. This work explores simulation based inference which relies on likelihood-to-evidence ratio estimation for the parameters of binary black holes mergers. We build a neural network modeling this ratio and use it in place of the simulator allowing to perform parameter inference in few minutes. The performances are assessed on both gravitational wave generated by the simulator and emitted by real black holes.
Fichier(s)
Document(s)
Annexe(s)
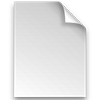

Description: Examples of inference of the masses performed on simulated gravitational waves. The 50% and 90% credible intervals are derived. The blue dot represents the maximum a posteriori estimator and the orange star the true parameters.
Taille: 192.54 kB
Format: Adobe PDF
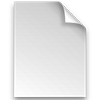

Description: Examples of inference of the distance and inclination performed on simulated gravitational waves. The 50% and 90% credible intervals are derived. The blue dot represents the maximum a posteriori estimator and the orange star the true parameters.
Taille: 299.07 kB
Format: Adobe PDF
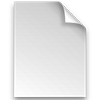

Description: Examples of inference of the effective spin and mass ratio performed on simulated gravitational waves. The 50% and 90% credible intervals are derived. The blue dot represents the maximum a posteriori estimator and the orange star the true parameters.
Taille: 235.01 kB
Format: Adobe PDF
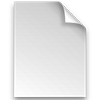

Description: Examples of inference of the sky position performed on simulated gravitational waves. The 50% and 90% credible intervals are derived. The blue dot represents the maximum a posteriori estimator and the orange star the true parameters.
Taille: 271.62 kB
Format: Adobe PDF
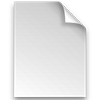

Description: Inference on the masses for the GW150914 signal. MCMC stands for Markov Chain Monte-Carlo and LFI for Likelihood-Free Inference.
Taille: 503.92 kB
Format: Adobe PDF
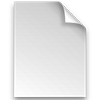

Description: Inference on the distance and the inclination for the GW150914 signal. MCMC stands for Markov Chain Monte-Carlo and LFI for Likelihood-Free Inference.
Taille: 832.77 kB
Format: Adobe PDF
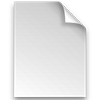

Description: Inference on the effective spin and the mass ratio for the GW150914 signal. MCMC stands for Markov Chain Monte-Carlo and LFI for Likelihood-Free Inference.
Taille: 484.74 kB
Format: Adobe PDF
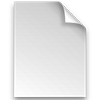

Description: Inference on the sky position for the GW150914 signal. MCMC stands for Markov Chain Monte-Carlo and LFI for Likelihood-Free Inference.
Taille: 64 kB
Format: Adobe PDF
Citer ce mémoire
L'Université de Liège ne garantit pas la qualité scientifique de ces travaux d'étudiants ni l'exactitude de l'ensemble des informations qu'ils contiennent.