Master's Thesis : Evaluating outcome following knee arthroplasty using inertial measurement units
Cantamessa, Astrid
Promotor(s) :
Geris, Liesbet
Date of defense : 7-Sep-2020/9-Sep-2020 • Permalink : http://hdl.handle.net/2268.2/10555
Details
Title : | Master's Thesis : Evaluating outcome following knee arthroplasty using inertial measurement units |
Translated title : | [fr] Évaluation du résultat d'une arthroplastie de genou à l'aide de capteurs inertiels |
Author : | Cantamessa, Astrid ![]() |
Date of defense : | 7-Sep-2020/9-Sep-2020 |
Advisor(s) : | Geris, Liesbet ![]() |
Committee's member(s) : | Ruffoni, Davide ![]() Schwartz, Cédric ![]() Monk, Paul Besier, Thor |
Language : | English |
Number of pages : | 98 |
Keywords : | [en] machine learning [en] inertial measurement units [en] knee arthroplasty [en] knee kinematics [en] patient monitoring [en] gait analysis [en] surgical outcome |
Discipline(s) : | Engineering, computing & technology > Multidisciplinary, general & others |
Research unit : | Auckland Bioengineering Institute |
Institution(s) : | Université de Liège, Liège, Belgique |
Degree: | Master en ingénieur civil biomédical, à finalité spécialisée |
Faculty: | Master thesis of the Faculté des Sciences appliquées |
Abstract
[en] Osteoarthritis is a frequent and debilitating disease whose burden is set to increase given our ageing population. Arthroplasty is the only curative treatment for end-stage arthritis and can greatly improve joint function, control pain and enhance quality of life. However, surgery is only part of the picture, and ensuring successful outcomes requires both extensive tailored physiotherapy and close patient monitoring for complications. Currently, patient reported outcome measures (PROMs) are used with minimal clinical follow-up. Not only does this allow for limited opportunities to assess postoperative function, but PROMs are also inherently subjective. As such, the orthopaedic clinic lacks of quantitative information with which to actively monitor a patient’s progress. In addition, due to resource limitations, it struggles to closely monitor patients during the first six weeks following surgery, a key period for ensuring adequate long-term joint function. Inertial measurement units (IMUs) provide an opportunity to objectively measure important biomechanical gait variables in both clinic and home settings. This allows clinicians and physiotherapists to remotely monitor patients through cloud-computing technologies.
The aim of this thesis, which is part of a larger research project at the Auckland Bioengineering Institute (Auckland, New Zealand), is to develop and assess a new workflow based on machine learning algorithm to quantitatively evaluate joint function during walking gait of patients following knee arthroplasty using only two ankle-worn IMUs. To evaluate this algorithm, predictions of joint kinematics were compared to ‘ground truth’ joint kinematics recorded from optical motion capture.
Twelve patients undergoing knee arthroplasty were recruited. They participated in two gait sessions before and around six weeks after their surgery during which optical marker trajectories and acceleration and angular velocity from IMUs were recorded. However, in view of the issues encountered with their quantity and quality, two other datasets, previously collected for other studies, were also exploited. One involved ten healthy volunteers performing treadmill walking and the second was composed of four overground walking healthy participants.
Two types of models were generated and evaluated: a personalised model, trained on a portion of a subject’s data and predicting the remaining part, and a generalised model, trained on every individual of the cohort but one used for prediction. Moreover, a sensitivity analysis was performed to select the most optimal combination of parameters and data processing ways. Our method enables to predict knee kinematics with more than 95\% accuracy for personalised models. This also holds for the treadmill generalised model. However, the poor performance of the overground generalised model was due to limited number of steps per person which could not capture the variability within the dataset.
The continuation of this study should increase the patient dataset and include other motions than walking. Moreover, information obtained about the outcome recorded in patients’ environment will be contrasted with other metrics (PROMs, range of motion) collected during their clinical follow-up. Ultimately, this may help clinicians to identify potential complications during recovery and provide the opportunity for early intervention.
File(s)
Document(s)
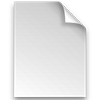

Description:
Size: 14.62 MB
Format: Adobe PDF
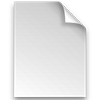

Description: -
Size: 14.62 MB
Format: Adobe PDF
Annexe(s)
Cite this master thesis
The University of Liège does not guarantee the scientific quality of these students' works or the accuracy of all the information they contain.