Master thesis : A Causal Approach to Marketing Mix Modelling through Bayesian Networks
Baré, Alexandre
Promotor(s) :
Louppe, Gilles
Date of defense : 27-Jun-2022/28-Jun-2022 • Permalink : http://hdl.handle.net/2268.2/15694
Details
Title : | Master thesis : A Causal Approach to Marketing Mix Modelling through Bayesian Networks |
Author : | Baré, Alexandre ![]() |
Date of defense : | 27-Jun-2022/28-Jun-2022 |
Advisor(s) : | Louppe, Gilles ![]() |
Committee's member(s) : | Wehenkel, Louis ![]() Sacré, Pierre ![]() Mohan, Siddharth |
Language : | English |
Discipline(s) : | Engineering, computing & technology > Computer science |
Target public : | Researchers Professionals of domain Student |
Institution(s) : | Université de Liège, Liège, Belgique |
Degree: | Master : ingénieur civil en science des données, à finalité spécialisée |
Faculty: | Master thesis of the Faculté des Sciences appliquées |
Abstract
[en] This thesis deals with the shortcomings of traditional Marketing Mix Models by proposing a new approach based on causality. As in most Machine Learning applications, it is common to rely on black-box models that can be hard to trust and which often translate into a longer adoption time by the industry. We argue that modelling performance is strengthened by combining raw data with business knowledge from domain experts. The alternative methodology that is thus here investigated relies on a proper causal process clearly identifying the cause-to-effect relationships in a Directed Acyclic Graph that describes the journeys from external and marketing factors to sales. For this purpose, we leverage the recent advances in Structure Learning for Causal Discovery and the power of Do-Calculus to build a framework for Marketing Return on Investment projects. Causal discovery allows for a semi-automated construction of Bayesian Networks and Do-Calculus enables interventional causal inference. The ultimate goal is to advise in a transparent way on an optimal mix of marketing channels.
File(s)
Document(s)
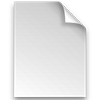

Description:
Size: 15.62 MB
Format: Adobe PDF
Cite this master thesis
The University of Liège does not guarantee the scientific quality of these students' works or the accuracy of all the information they contain.