Master thesis and internship[BR]- Master's thesis : Ship detection from SAR imaging and machine learning techniques[BR]- Integration internship : Centre Spatial de Liège
Cappart, Alice
Promoteur(s) :
Habraken, Serge
Date de soutenance : 27-jan-2023 • URL permanente : http://hdl.handle.net/2268.2/17086
Détails
Titre : | Master thesis and internship[BR]- Master's thesis : Ship detection from SAR imaging and machine learning techniques[BR]- Integration internship : Centre Spatial de Liège |
Auteur : | Cappart, Alice ![]() |
Date de soutenance : | 27-jan-2023 |
Promoteur(s) : | Habraken, Serge ![]() |
Membre(s) du jury : | Kirkove, Murielle ![]() Glaude, Quentin ![]() Van Droogenbroeck, Marc ![]() |
Langue : | Anglais |
Mots-clés : | [en] Machine Learning [en] Ship Detection [en] SAR |
Discipline(s) : | Physique, chimie, mathématiques & sciences de la terre > Aérospatiale, astronomie & astrophysique |
Centre(s) de recherche : | Centre Spatial de Liège |
Public cible : | Chercheurs Professionnels du domaine Etudiants |
Institution(s) : | Université de Liège, Liège, Belgique |
Diplôme : | Master en ingénieur civil en aérospatiale, à finalité spécialisée en "aerospace engineering" |
Faculté : | Mémoires de la Faculté des Sciences appliquées |
Résumé
[en] Human activities at sea have been not only impacting the maritime environment for years
but also human development and society. Marine monitoring is one of the main thematic
areas addressed by the Copernicus European Union’s Earth Obesrvation Programme.
In particular, Sentinel-1 uses synthetic aperture radar (SAR) technology that provides
global coverage and high-resolution images of the ocean surface, allowing monitoring
of the position of ships at sea which may be useful to track illegal activities, such as
fishing or oil spills, or for safety and security. Using machine learning algorithms allows
to automatically analyze SAR images and supplies ship detection with better accuracy
and efficiency. The present paper aims at evaluating the relevance of LS-SSDD-v1.0,
i.e Large-Scale SAR Ship Detection Dataset-v1.0 Large, for training machine learning
algorithms for ship detection from Sentinel-1 SAR images, and more particularly for
small ship detection. To conduct this work effectively, the former dataset is used to train
and evaluate RetinaNet and Faster R-CNN baseline models using common classification
performance metrics. As a bonus, the Hungarian algorithm is implemented to solve the
assignment problem between SAR ship predictions and AIS messages. The final selected
model is applied on a SAR image of the Belgian coast and the detection is compared to the
AIS messages of the region of interest at the same time of the Sentinel-1 acquisition. In
conclusion, the comparison between both ship positions as well as the model performance
results suggest that LS-SSDD-v1.0 is a relevant database for this master’s thesis subject
but may still need to be augmented. A larger number of predicted ships than AIS messages
also implies either illegal ships or a lack of received AIS signals.
Fichier(s)
Document(s)
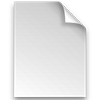

Description:
Taille: 55.62 MB
Format: Adobe PDF
Annexe(s)
Citer ce mémoire
L'Université de Liège ne garantit pas la qualité scientifique de ces travaux d'étudiants ni l'exactitude de l'ensemble des informations qu'ils contiennent.