Response surface-based prediction of ship resistance in shallow water conditions
Gamaralalage, Ravindu Udayanga Senavirathna
Promoteur(s) :
Rigo, Philippe
Date de soutenance : 27-jan-2023 • URL permanente : http://hdl.handle.net/2268.2/18068
Détails
Titre : | Response surface-based prediction of ship resistance in shallow water conditions |
Titre traduit : | [fr] Prévision de la résistance des navires en eaux peu profondes basée sur la surface de réponse |
Auteur : | Gamaralalage, Ravindu Udayanga Senavirathna ![]() |
Date de soutenance : | 27-jan-2023 |
Promoteur(s) : | Rigo, Philippe ![]() |
Langue : | Anglais |
Nombre de pages : | 117 |
Mots-clés : | [en] shallow water hydrodynamics, machine learning, Kriging, ANN, surrogate model |
Discipline(s) : | Ingénierie, informatique & technologie > Multidisciplinaire, généralités & autres |
Public cible : | Chercheurs Professionnels du domaine Etudiants |
Institution(s) : | Université de Liège, Liège, Belgique |
Diplôme : | Master : ingénieur civil mécanicien, à finalité spécialisée en "Advanced Ship Design" |
Faculté : | Mémoires de la Faculté des Sciences appliquées |
Résumé
[en] Ships experience increasing hydrodynamic resistance as the depth of the water decreases. This difference in resistance between shallow and deep water is termed as the added resistance in shallow water that exhibits a non-linear behaviour with respect to operational parameters such as water depth to ship draft ratio $(h/T)$, velocity of the ship $(v)$, and depth-based Froude number $(Frh)$. A comprehensive development framework is proposed to generate a surrogate model to estimate shallow water added resistance on a modern chemical oil tanker. The developed model was proven to provide cheaper and faster resistance predictions with acceptable error margins, as compared to the computationally/capitally expensive CFD simulations and model tests.
Several surrogate models were built using Kriging and Artificial Neural Networks (ANN) methods with a dataset containing $\apr556$ combinations of water depth $(h)$, draft $(T)$, ship velocity $(v)$, and their corresponding added resistance values $(\Delta F_T)$ alculated with RANSE-based OpenFOAM CFD simulations. At their base states, 88\% and 64\% predictive accuracies were demonstrated by the best Kriging and ANN models with respect to the $r^2$ matric. After tuning the hyperparameters, the accuracy of the best ANN model went up to 85\%. The same hyperparameter tuning process resulted in a Kriging model with 97.6\% prediction accuracy, which was identified as the ``best performer''. A fully-automated, iterative refinement loop was introduced to further improve the local uncertainties. The finalized iterative loop integrated a squared error and LHS-based adaptive sampling strategy to introduce further information to the local areas with the highest prediction uncertainty at each iterative step. A 0.5\% performance improvement was seen on the ``best performer'' surrogate model after a 20-iteration test run of the loop.
The ``best performer'' surrogate model is nominated to be integrated into the MARIDATA decision support system, a cutting-edge initiative funded by the German Federal Ministry of Economic Affairs and Climate Action, which is aimed at enhancing the sustainability and efficiency of ship operations. Moreover, the fully open-source, Python-based modelling framework developed is denoted to act as a basis to create surrogate models for shallow water resistance prediction for ships other than the chemical oil tanker considered in this project. With minor improvements, this use case can be extended to predict other ship operational parameters.
Fichier(s)
Document(s)
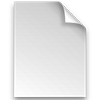

Description:
Taille: 38.56 MB
Format: Adobe PDF
Citer ce mémoire
L'Université de Liège ne garantit pas la qualité scientifique de ces travaux d'étudiants ni l'exactitude de l'ensemble des informations qu'ils contiennent.