Design and Performance Analysis of a Neuromodulable Spiking Recurrent Cell in the Context of Supervised Learning
Bernard, Aurélien
Promotor(s) :
Drion, Guillaume
Date of defense : 4-Sep-2023/5-Sep-2023 • Permalink : http://hdl.handle.net/2268.2/18193
Details
Title : | Design and Performance Analysis of a Neuromodulable Spiking Recurrent Cell in the Context of Supervised Learning |
Author : | Bernard, Aurélien ![]() |
Date of defense : | 4-Sep-2023/5-Sep-2023 |
Advisor(s) : | Drion, Guillaume ![]() |
Committee's member(s) : | Ernst, Damien ![]() Sacré, Pierre ![]() Franci, Alessio ![]() |
Language : | English |
Discipline(s) : | Engineering, computing & technology > Electrical & electronics engineering |
Target public : | Researchers Professionals of domain Student |
Institution(s) : | Université de Liège, Liège, Belgique |
Degree: | Master : ingénieur civil électricien, à finalité spécialisée en "signal processing and intelligent robotics" |
Faculty: | Master thesis of the Faculté des Sciences appliquées |
Abstract
[en] From the desire to understand how neurons work and how we learn, neuroscientists from early 1900 to the 1950s proposed increasingly more complex neuron models. However, no learning rule seemed to have a satisfactory performance until the invention of back-propagation which uses optimisation and calculus to optimise the performance at a certain task. This new learning rule required neural networks to steer away from highly non-linear biological neuron models to ones with continuous properties that allow them to work. Machine Learning researchers favoured this new technique and have obtained increasingly better results with increasingly larger networks, large amounts of data, more computing power necessary to use them, and inevitably, higher power requirements. In an attempt to solve the shortcomings of modern artificial neural networks, the machine learning community is looking again into bio-inspired spiking neural networks and new learning rules that would perform as well as backpropagation. To this date, these attempts have had varying levels of success but have all fallen short of the modern standards of machine learning. However, a recent contribution has proposed a new way of creating and training spiking neural networks by re-designing classical recurrent neural network cells to force them to exhibit spiking behaviour. This allows the use of backpropagation without the need for approximations or adaptations as other spiking neural network training methods have proposed in the past. In this work, we analyse the proposed spiking recurrent cell from a nonlinear systems dynamics perspective and observe some of its shortcomings. Then, we propose two new cells that extend and reduce some of the previously observed issues. Namely, we extend the cell by introducing neuromodulation capabilities to have 3 distinct types of excitability through the addition of a single parameter, and with the second proposed cell, we extend further its capabilities by introducing bursting behaviour. Finally, we perform tests on small neural networks composed of 42 neurons based on the former cell on the MNIST benchmark coded in spikes through latency, and rate coding. In the experiments, we first modulate the neurons to fixed firing types and analyse the performance and behaviour of the network on the 2 tasks. We then randomly initialise the modulation through the network and set it as a learnable parameter for the same tasks. In these tests, we have achieved 91\% accuracy which is close to state-of-the-art performance on SNNs that contain more than 100 times the number of neurons. Furthermore, we have observed that in the current setup, the network does not use a heterogeneity of firing types in the network to obtain the best results. Instead, it converges to one of the newly introduced firing types.
Concisely, the main goal of this work is to introduce neuromodulation to a spiking neural network cell that learns through direct backpropagation, and show that modulation can also be learned through this same learning algorithm to obtain satisfactory results.
File(s)
Document(s)
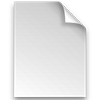

Description: Thesis summary
Size: 2.91 MB
Format: Adobe PDF
Cite this master thesis
The University of Liège does not guarantee the scientific quality of these students' works or the accuracy of all the information they contain.