Machine learning methods to improve insulin sensitivity forecasting in glycemic control
Frambach, Julien
Promotor(s) :
Desaive, Thomas
Date of defense : 4-Sep-2023/5-Sep-2023 • Permalink : http://hdl.handle.net/2268.2/18224
Details
Title : | Machine learning methods to improve insulin sensitivity forecasting in glycemic control |
Author : | Frambach, Julien ![]() |
Date of defense : | 4-Sep-2023/5-Sep-2023 |
Advisor(s) : | Desaive, Thomas ![]() |
Committee's member(s) : | Uyttendaele, Vincent ![]() Chase, Geoff Louppe, Gilles ![]() |
Language : | English |
Keywords : | [en] Machine learning, Glycemic control, insulin sensitivity |
Discipline(s) : | Engineering, computing & technology > Civil engineering |
Target public : | Professionals of domain Student |
Institution(s) : | Université de Liège, Liège, Belgique |
Degree: | Master : ingénieur civil électricien, à finalité spécialisée en "electronic systems and devices" |
Faculty: | Master thesis of the Faculté des Sciences appliquées |
Abstract
[en] Stress-induced hyperglycemia is a common complication among critically ill patients in the in-
tensive care unit. This health condition poses significant dangers, as it can increase the risks
of morbidity and mortality. Glycaemic control has been shown beneficial for these patients but
hard to achieve safely and consistently. STAR is a patient-specific, model-based glucose control
protocol framework successfully providing safe and effective blood glucose control within nor-
moglyceic range using insulin and nutrition as treatments. The STAR protocol has undergone
clinical validation, a challenging task due to glycemic variability and the need to prevent hypo-
glycemic episodes.
Currently, the STAR protocol uses a kernel density approach to predict the 5th and 95th
percentiles of the insulin sensitivity within 1-3 hourly intervals. Forecasting insulin sensitivity is
an important criterion of the STAR protocol to give the best treatment for the patient. Specif-
ically, the STAR 3D model leverages the past two values to predict the distributions of insulin
sensitivity and recommend the optimal treatment based on predicted risks. However, there is
an opportunity to enhance the current model by incorporating machine learning techniques.
This thesis explores the application of various machine learning models using quantile regres-
sion to forecast these percentiles. The aim is to improve prediction accuracy and subsequently
enhance patient safety within the intensive care unit environment.
The findings from these machine learning models highlight the challenge of achieving sub-
stantial prediction improvements using only two input features. The solution lies in increasing
the number of features, thereby enhancing prediction performance and potentially reducing the
occurrence of both hypoglycemic and hyperglycemic episodes among patients.
File(s)
Document(s)
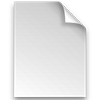

Description: Correct version
Size: 4.09 MB
Format: Adobe PDF
Annexe(s)
Cite this master thesis
The University of Liège does not guarantee the scientific quality of these students' works or the accuracy of all the information they contain.