Master Thesis : Music Source Separation with Neural Networks
Laurent, Martin
Promotor(s) :
Louppe, Gilles
Date of defense : 4-Sep-2023/5-Sep-2023 • Permalink : http://hdl.handle.net/2268.2/18349
Details
Title : | Master Thesis : Music Source Separation with Neural Networks |
Translated title : | [fr] Séparation de Source Musicales avec des Réseaux de Neurones Profonds |
Author : | Laurent, Martin ![]() |
Date of defense : | 4-Sep-2023/5-Sep-2023 |
Advisor(s) : | Louppe, Gilles ![]() |
Committee's member(s) : | Geurts, Pierre ![]() Sacré, Pierre ![]() |
Language : | English |
Number of pages : | 65 |
Discipline(s) : | Engineering, computing & technology > Civil engineering |
Institution(s) : | Université de Liège, Liège, Belgique |
Degree: | Master : ingénieur civil en science des données, à finalité spécialisée |
Faculty: | Master thesis of the Faculté des Sciences appliquées |
Abstract
[fr] Music source separation (MSS) involves extracting the individual instrument's audio sources from a mixed music signal and is known to be a very challenging problem with a long history of scientific activity. In the last decade, the field has known significant progress thanks to the emergence of Deep Learning, with deep neural network architectures achieving state-of-the-art performances. In this master thesis we explore how Deep Learning is applied to the problem of MSS and examine some of the best models to date. We then attempted to reproduce one of those models which applies the traditional Short Time Fourier Transform to the input signal. We finally explore a potential of an alternative transform, the Short Time Discrete Cosine Transform, for this architecture which brings the possibility of achieving the same results while reducing drastically the complexity and training time of the model.
File(s)
Document(s)
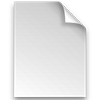

Description:
Size: 13.29 MB
Format: Adobe PDF
Cite this master thesis
The University of Liège does not guarantee the scientific quality of these students' works or the accuracy of all the information they contain.