Does the real economy still forecast stock returns? Evidence in an age of digital economy and crises
Lorant, Marie
Promotor(s) :
Hambuckers, Julien
Date of defense : 2-Sep-2024/7-Sep-2024 • Permalink : http://hdl.handle.net/2268.2/21176
Details
Title : | Does the real economy still forecast stock returns? Evidence in an age of digital economy and crises |
Author : | Lorant, Marie ![]() |
Date of defense : | 2-Sep-2024/7-Sep-2024 |
Advisor(s) : | Hambuckers, Julien ![]() |
Committee's member(s) : | Crucil, Romain ![]() |
Language : | English |
Number of pages : | 100 |
Keywords : | [fr] Stock market [fr] monthly return forecasting [fr] real economy [fr] combination forecast [fr] US |
Discipline(s) : | Business & economic sciences > Finance |
Target public : | Professionals of domain Student |
Institution(s) : | Université de Liège, Liège, Belgique |
Degree: | Master en ingénieur de gestion, à finalité spécialisée en Financial Engineering |
Faculty: | Master thesis of the HEC-Ecole de gestion de l'Université de Liège |
Abstract
[en] This master's thesis investigates the potential link between the real economy and the predictability of equity returns. The aim of the study is to analyze the conclusions reached by Rapach et al. in 2010, in a more recent context. They found out 2 things. First, the real economy, represented through macroeconomic variables, is linked to the stock returns. Second, the combination methods they developped are capable of outperforming a simple historical average. The world having changed, the analysis is carried out over the post-paper years, i.e. after 2010. Our analysis is based on the prediction of monthly equity returns, based on the S&P500 index during 4 out-sample periods. The creation of the individual forecasting models use OLS regression as well as machine learning methods. The forecasting combined models used are derived from the methods used by Rapach et al. Therefore, 3 combined methods are used within each out-sample periods. The greatest contribution to the literature is the fact that we were able to define a link between the real economy and the financial market, via equity returns. However, the link established is weaker than that observed pre-paper, i.e. before 2010. Moreover, the combination methods used in this thesis are only economically interesting half of the time. Although the results may be satisfactory, they are less conclusive than in 2010. Another important contribution of this thesis is the fact that machine learning methods, although very flexible, are not always the most efficient. While these findings are interesting, the financial literature agrees that financial market prediction remains a highly complex subject. As a result, there is still much room for improvement in the forecast ability of models.
File(s)
Document(s)
Annexe(s)
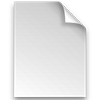

Description:
Size: 49.16 kB
Format: Unknown
Cite this master thesis
The University of Liège does not guarantee the scientific quality of these students' works or the accuracy of all the information they contain.