CraterNet : a Fully Convolutional Neural Network for Lunar Crater Detection Based on Remotely Sensed Data
Glaude, Quentin
Promotor(s) :
Cornet, Yves
Date of defense : 26-Jun-2017/27-Jun-2017 • Permalink : http://hdl.handle.net/2268.2/2486
Details
Title : | CraterNet : a Fully Convolutional Neural Network for Lunar Crater Detection Based on Remotely Sensed Data |
Translated title : | [fr] CraterNet : un réseau neuronal entièrement convolutif pour la détection de cratères lunaires sur base de produits issus de la télédétection |
Author : | Glaude, Quentin ![]() |
Date of defense : | 26-Jun-2017/27-Jun-2017 |
Advisor(s) : | Cornet, Yves ![]() |
Committee's member(s) : | Demoulin, Alain ![]() Geurts, Pierre ![]() Van Droogenbroeck, Marc ![]() |
Language : | English |
Number of pages : | 159 |
Keywords : | [en] Fully Convolutional Networks [en] Crater Detection [en] Deep Learning |
Discipline(s) : | Engineering, computing & technology > Computer science Physical, chemical, mathematical & earth Sciences > Earth sciences & physical geography |
Target public : | Researchers Professionals of domain Student |
Institution(s) : | Université de Liège, Liège, Belgique |
Degree: | Master en sciences géographiques, orientation géomatique et géométrologie, à finalité spécialisée |
Faculty: | Master thesis of the Faculté des Sciences |
Abstract
[en] In this master thesis, we propose a novel approach to detect lunar craters using new deep learning advances. The architecture of the model employed is a fully convolutional neural network that uses the freely available remotly sensed data from the «Lunar Reconnaissance Orbiter» space probe. In this brief, we discuss the methodology, the choices made and the evaluation of the model with different visual and quantitative results in addition to the source code.
File(s)
Document(s)
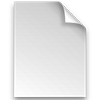

Description: CraterNet : a Fully Convolutional Neural Network for Crater Detection on Lunar Remotely Sensed Data
Size: 22.86 MB
Format: Adobe PDF
Annexe(s)
Cite this master thesis
All documents available on MatheO are protected by copyright and subject to the usual rules for fair use.
The University of Liège does not guarantee the scientific quality of these students' works or the accuracy of all the information they contain.
The University of Liège does not guarantee the scientific quality of these students' works or the accuracy of all the information they contain.