Predicting the houseprice index using sentiment analysis and machine learning
Hajdari, Bledi
Promotor(s) :
Ittoo, Ashwin
Date of defense : 19-Jun-2020/23-Jun-2020 • Permalink : http://hdl.handle.net/2268.2/8813
Details
Title : | Predicting the houseprice index using sentiment analysis and machine learning |
Translated title : | [fr] Prédire l'indice des prix de l'immobilier en utilisant l'analyse des sentiments et l'apprentissage automatique |
Author : | Hajdari, Bledi ![]() |
Date of defense : | 19-Jun-2020/23-Jun-2020 |
Advisor(s) : | Ittoo, Ashwin ![]() |
Committee's member(s) : | Heins, Eddy ![]() Schyns, Michael ![]() Lamest, Markus |
Language : | English |
Keywords : | [en] real estate, sentiment analysis, machine learning |
Discipline(s) : | Engineering, computing & technology > Computer science |
Target public : | Researchers Professionals of domain |
Institution(s) : | Université de Liège, Liège, Belgique |
Degree: | Master en ingénieur de gestion, à finalité spécialisée en Supply Chain Management and Business Analytics |
Faculty: | Master thesis of the HEC-Ecole de gestion de l'Université de Liège |
Abstract
[en] The 2008 financial crisis showed that economists seem to be far from a complete understanding of the real estate market fluctuations. There is often a strong gap between the variations of fundamental economic and demographic factors and the actual housing price changes.. An alternative approach to the study real estate market fluctuations, called Behavioral Real Estate, emerged from the evidence of unexplained volatility and market inefficiency. It deviates from the traditional way of studying the real estate market by explaining changes in house prices with traditional factors like inflation, interest rates and construction costs.
This study focuses on forecasting the housing market and seeks to provide new evidence on the role of sentiment in driving house prices, which, to date, is a fairly underestimated approach. The difficulty in measuring the sentiment regarding the real estate market has made it really challenging to explain volatility in the housing market, despite clear evidence of psychological factors in explaining price fluctuations. Throughout the thesis, we followed the hypothesis that public opinion on social media has an impact on the behavior of the real estate market participants and subsequently affects future price trends.
To test our hypothesis, we have trained a model with economic variables to predict the London House Price Index (HPI) using recurrent neural networks. Then, we have built a tweet-based sentiment index for the London housing market and incorporated it as a feature in our initial model. The model trained with economic and sentiment data performs better (MAE = 0.8315 and RMSE = 1.0358) than the one with economic data alone (MAE = 0.9012 and RMSE = 1.1701). Moreover, the permutation feature importance showed that the sentiment index is a significant feature in our predictive model.
These results confirm our initial beliefs that the incorporation of a sentiment indicator should improve the overall performance of the model. This implies that real estate forecasters, policymakers and professional investors should consider incorporating this free data into their market predictions or when deciding for future investments.
File(s)
Document(s)
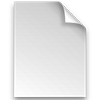

Description:
Size: 6.26 MB
Format: Adobe PDF
Cite this master thesis
The University of Liège does not guarantee the scientific quality of these students' works or the accuracy of all the information they contain.