Sales forecasting using statistical and machine learning models: the case of HEXPOL
Loewenau, Hendrik
Promotor(s) :
Dumont, Morgane
Date of defense : 10-Jun-2024/22-Jun-2024 • Permalink : http://hdl.handle.net/2268.2/19634
Details
Title : | Sales forecasting using statistical and machine learning models: the case of HEXPOL |
Author : | Loewenau, Hendrik ![]() |
Date of defense : | 10-Jun-2024/22-Jun-2024 |
Advisor(s) : | Dumont, Morgane ![]() |
Committee's member(s) : | Aerts, Stéphanie ![]() Panckert, Daniel |
Language : | English |
Number of pages : | 65 |
Keywords : | [en] B2B [en] Machine learning [en] Sales forecasting [en] statistics |
Discipline(s) : | Business & economic sciences > Quantitative methods in economics & management |
Institution(s) : | Université de Liège, Liège, Belgique |
Degree: | Master en sciences de gestion (Horaire décalé) |
Faculty: | Master thesis of the HEC-Ecole de gestion de l'Université de Liège |
Abstract
[en] Sales forecasting occupies an important place in manufacturing companies, especially in the B2B sector. It can help organisations optimise their supply chain management, resource allocation and target setting. There has been some development made in the different models and new techniques are derived from existing ones. However, there are still some important challenges and limitations in providing accurate forecasts.
This thesis aims to answer three main questions about which technique and model would be adequate to be used in sales forecasting and whether external data should be included in the forecasting model and what kind of data. Furthermore, it discusses the different statistical and machine-learning model setups applied in search of the optimal outcome. The answers to these questions determine whether of this proof of concept is satisfactory or not. The conclusion determines if a statistical or machine-learning forecasting model could present an alternative or an extension to conventional judgemental forecasting.
The Prophet model which has been developed by the Facebook engineering team turned out to be the best-performing model, whereas the artificial neural network did not perform well based on the chosen evaluation metrics. To better understand the results and put them into context, they are visualised in the BI software “Tableau”.
Improvements in terms of accuracy and precision could still be made by extending the feature selection and taking more or different external datasets into consideration.
File(s)
Document(s)
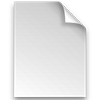

Description:
Size: 2.51 MB
Format: Adobe PDF
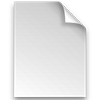

Description:
Size: 2.5 MB
Format: Adobe PDF
Cite this master thesis
The University of Liège does not guarantee the scientific quality of these students' works or the accuracy of all the information they contain.